Alphabet x use ai food production – Alphabet X uses AI for food production, revolutionizing everything from farm to fork. Imagine AI-powered systems optimizing irrigation, predicting crop diseases, and even personalizing food products to meet consumer demands. This isn’t science fiction; it’s the cutting edge of food tech, and Alphabet X is leading the charge. We’ll dive into their innovative projects, the technological hurdles, and the ethical considerations of this exciting, and sometimes controversial, field.
This exploration will cover Alphabet X’s specific initiatives, comparing their strategies with other tech giants. We’ll examine how AI enhances efficiency and safety in food processing, reduces waste, and contributes to a more sustainable food system. We’ll also address the ethical implications, including potential biases in algorithms and the impact on food security for diverse populations.
Alphabet X’s AI Initiatives in Food Production: Alphabet X Use Ai Food Production
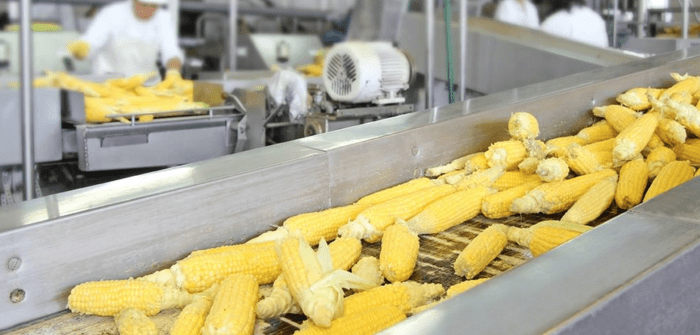
Source: emrspace.com
Alphabet X, the moonshot factory of Google’s parent company, Alphabet Inc., is quietly revolutionizing the food industry with its AI-powered innovations. While not as publicly visible as some of their other projects, their investments in this sector hold significant potential to address global food security challenges and optimize agricultural practices. This exploration delves into Alphabet X’s current AI projects within the food sector, their potential applications, the technological hurdles they face, and how their approach compares to other tech giants.
Alphabet X’s current AI investments in food production are largely focused on improving efficiency and sustainability throughout the entire food supply chain. While specific details are often kept confidential due to the experimental nature of many projects, reports suggest a strong emphasis on using machine learning to optimize crop yields, predict disease outbreaks, and reduce waste. This approach leverages the power of data analysis to improve decision-making at every stage, from planting seeds to delivering food to consumers.
AI Applications Across the Food Production Chain
AI’s potential applications within the food production chain are vast and transformative. From precision agriculture using drones and sensor data to predict optimal planting times and fertilizer application, to AI-powered robots automating harvesting and sorting processes, the technology offers significant opportunities for increased efficiency and reduced labor costs. Furthermore, AI can enhance food safety by detecting contamination early on and improving quality control measures. In the distribution and retail stages, AI-driven logistics can optimize delivery routes, reduce spoilage, and minimize food waste. Consider the impact of predicting consumer demand accurately – less food would be produced and wasted, leading to significant economic and environmental benefits.
Technological Challenges in Deploying AI for Food Production
Despite the potential, deploying AI in food production faces significant technological challenges. One major hurdle is the sheer volume and complexity of data involved. Integrating data from diverse sources – weather patterns, soil conditions, crop yields, market prices – requires robust and scalable data infrastructure. Another challenge lies in the need for robust and reliable AI models that can handle the variability inherent in agricultural systems. Unpredictable weather patterns, pest infestations, and soil variations can significantly impact model accuracy. Finally, the lack of standardized data formats and the need for interoperability between different AI systems pose further challenges.
Comparison with Other Tech Companies
Alphabet X’s approach to AI in food production differs from other tech companies in several key aspects. While companies like Microsoft and IBM are also investing heavily in agricultural AI, Alphabet X’s focus seems to be more on fundamental research and developing cutting-edge technologies. They are less focused on immediate commercial applications and more on long-term, potentially disruptive solutions. This approach contrasts with other companies that often prioritize near-term market opportunities and partnerships with established agricultural businesses. Alphabet X’s approach, while potentially riskier, could lead to more impactful and innovative solutions in the long run.
AI-Driven Optimization of Agricultural Practices
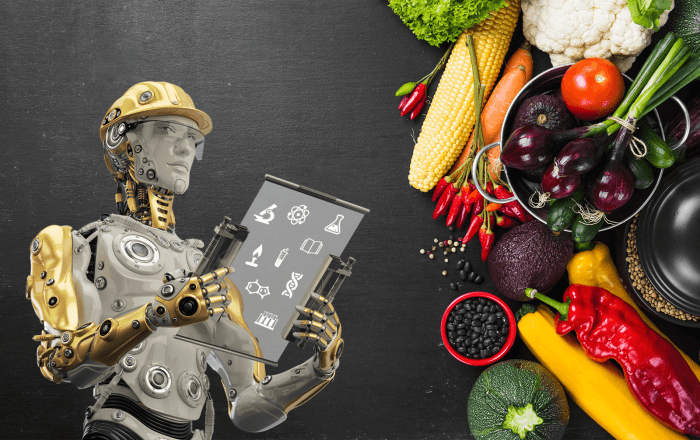
Source: elshohail.com
Forget dusty almanacs and guesswork – AI is revolutionizing farming, transforming it from a largely reactive process to a proactive, data-driven enterprise. By harnessing the power of machine learning and predictive analytics, we’re seeing unprecedented improvements in efficiency, yield, and sustainability across the agricultural landscape. This shift is not just about incremental improvements; it’s a fundamental reshaping of how we grow and manage our food supply.
AI is poised to solve some of agriculture’s most pressing challenges, from optimizing resource allocation to mitigating the impact of climate change. This involves sophisticated systems that analyze massive datasets, identifying patterns and predicting outcomes with remarkable accuracy. The result? Farmers can make informed decisions, minimizing waste and maximizing productivity, leading to a more resilient and efficient food system.
AI-Powered Irrigation and Fertilization
An AI-powered irrigation and fertilization system would leverage sensors deployed throughout the field to gather real-time data on soil moisture, nutrient levels, and weather conditions. This data would be fed into a machine learning model trained to optimize irrigation schedules and fertilizer application based on the specific needs of the crop at any given time. For example, the system could identify areas experiencing drought stress and automatically adjust irrigation accordingly, preventing water waste and ensuring optimal crop growth. Similarly, it could pinpoint nutrient deficiencies and recommend precise fertilizer application, minimizing environmental impact and maximizing nutrient uptake by the plants. This precision approach contrasts sharply with traditional methods, which often rely on broad applications of water and fertilizer, leading to inefficiency and environmental damage.
AI-Driven Crop Disease and Pest Prediction and Prevention, Alphabet x use ai food production
AI can analyze images captured by drones or ground-based sensors to detect early signs of crop diseases and pest infestations. By identifying these issues before they become widespread, farmers can implement targeted interventions, such as applying specific pesticides or fungicides only where needed, reducing the overall use of chemicals and minimizing environmental harm. For instance, a system could identify a small patch of blight in a potato field and alert the farmer, allowing for prompt treatment before the disease spreads throughout the entire crop. This proactive approach is a significant departure from traditional methods, which often involve reactive measures only after a significant infestation has occurred. Early detection through AI translates to higher yields and healthier crops.
AI-Enhanced Crop Yield and Quality
AI can analyze vast datasets encompassing weather patterns, soil conditions, and historical yield data to predict optimal planting times, crop varieties, and harvesting schedules. This predictive capability enables farmers to make data-driven decisions that maximize yield and improve crop quality. For example, AI could predict the optimal planting date for a specific crop based on historical weather data and soil conditions, ensuring the crop has the best chance of reaching its full potential. Moreover, AI can analyze images of crops to assess their quality and identify defects, allowing for early sorting and grading, ultimately improving the overall quality of the produce reaching consumers.
AI’s Role in Reducing Food Waste
AI can play a crucial role in minimizing food waste throughout the agricultural supply chain. From optimizing harvesting and post-harvest handling to improving logistics and distribution, AI can help reduce losses at every stage. For example, AI-powered systems can predict optimal harvesting times based on ripeness levels, minimizing spoilage during transportation and storage. Similarly, AI can optimize transportation routes and storage conditions to minimize losses due to spoilage or damage. By improving efficiency and predictability throughout the supply chain, AI contributes to a more sustainable and less wasteful food system. Imagine a world where food waste is dramatically reduced, thanks to the insights provided by intelligent systems.
AI in Food Processing and Manufacturing
The food industry is ripe for disruption, and artificial intelligence is leading the charge. From farm to fork, AI is transforming how we produce, process, and distribute food, promising increased efficiency, improved safety, and a more personalized consumer experience. This section dives into the specific ways AI is revolutionizing food processing and manufacturing.
AI-Enhanced Efficiency and Safety in Food Processing Plants
AI is streamlining operations and bolstering safety protocols in food processing plants. Computer vision systems, for instance, can identify and remove defective products from assembly lines with far greater speed and accuracy than human inspectors, minimizing waste and ensuring consistent quality. Predictive maintenance algorithms, analyzing data from sensors on machinery, can anticipate equipment failures, preventing costly downtime and reducing the risk of accidents. Robotic systems guided by AI can perform repetitive and physically demanding tasks, improving worker safety and productivity. For example, a robotic arm equipped with AI could precisely and efficiently sort and pack produce, reducing the risk of human error and injury.
AI in Food Quality Control and Food Safety
Maintaining food safety and quality is paramount. AI plays a crucial role here. Advanced image recognition can detect contaminants or imperfections in food products that might be missed by the human eye, ensuring only safe and high-quality products reach consumers. AI-powered systems can also analyze large datasets of environmental and production data to identify potential sources of contamination and predict outbreaks, allowing for proactive intervention and prevention. Furthermore, blockchain technology, often integrated with AI, provides enhanced traceability throughout the supply chain, facilitating rapid identification and removal of contaminated products in case of an outbreak. This enhanced transparency builds consumer trust and strengthens food safety management.
AI-Powered System for Optimizing Food Packaging and Distribution
An AI-powered system for optimizing food packaging and distribution could leverage machine learning algorithms to analyze historical sales data, consumer preferences, and real-time demand to predict optimal inventory levels and distribution routes. This predictive capability minimizes waste from spoilage or overstocking and ensures products reach consumers efficiently and timely. The system could also optimize packaging design, considering factors like material costs, environmental impact, and shelf life to maximize efficiency and sustainability. For example, an AI could analyze data to determine the ideal size and type of packaging for a specific product, minimizing material waste and transportation costs.
AI-Driven Personalization of Food Products
AI enables the creation of personalized food products tailored to individual consumer preferences and dietary needs. By analyzing consumer data such as purchase history, health information, and social media activity, AI can identify patterns and predict future demand. This allows food manufacturers to develop customized products, catering to specific tastes, allergies, or dietary restrictions. For instance, a company could offer personalized meal plans based on individual nutritional requirements, generated by an AI that considers factors like age, activity level, and health goals. This level of personalization enhances customer satisfaction and loyalty.
Comparison of AI-Powered Food Processing Solutions
Solution | Enhanced Efficiency | Improved Safety | Predictive Analytics |
---|---|---|---|
Vision-based defect detection | Automated defect identification and removal, reducing waste and improving throughput. | Minimizes human error in inspection, reducing risk of contaminated products reaching consumers. | Predicts potential equipment failures, enabling proactive maintenance. |
AI-powered robotics | Automates repetitive tasks, increasing productivity and reducing labor costs. | Reduces risk of workplace injuries associated with manual labor. | Predicts optimal robot deployment based on workload and production demands. |
Predictive maintenance platform | Reduces downtime by predicting equipment failures, allowing for scheduled maintenance. | Minimizes risk of accidents caused by equipment malfunction. | Provides insights into equipment performance, optimizing maintenance schedules and resource allocation. |
AI and the Future of Food Sustainability
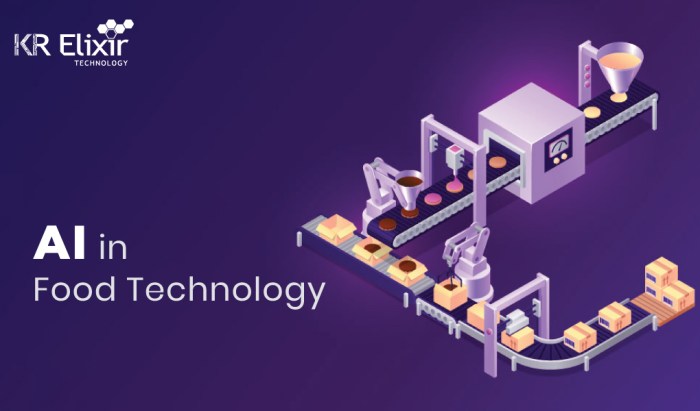
Source: krelixir.com
Alphabet X’s foray into AI-powered food production is pretty wild, right? Thinking about optimizing harvests and minimizing waste is one thing, but the tech world’s full of unexpected glitches; remember that crazy iphone x bug affecting Suica users in Japan ? It shows even the most advanced tech can have unforeseen consequences, highlighting the need for robust testing in all sectors, including the ambitious goal of AI-driven food systems.
The looming global food crisis demands innovative solutions, and artificial intelligence (AI) is emerging as a powerful tool to revolutionize food production and distribution, paving the way for a more sustainable and equitable food system. AI’s potential extends far beyond increased yields; it offers a pathway to minimize environmental impact, enhance resource efficiency, and improve food access for vulnerable populations.
AI can significantly contribute to more sustainable food production practices by optimizing resource allocation, reducing waste, and improving efficiency across the entire food supply chain. This involves precision agriculture, predictive analytics for crop management, and AI-powered solutions for reducing food loss and waste. The ultimate goal is to produce more food with fewer resources and less environmental damage.
AI’s Role in Reducing the Environmental Impact of Food Production
AI-driven precision agriculture offers substantial environmental benefits compared to traditional farming methods. For example, AI-powered systems can optimize irrigation by analyzing soil moisture levels and weather patterns, reducing water waste significantly. Similarly, AI can optimize fertilizer application, minimizing nutrient runoff and its associated environmental damage, such as eutrophication of waterways. Furthermore, AI-powered drones and sensors can monitor crop health, allowing for early detection of pests and diseases, reducing the need for broad-spectrum pesticides. This targeted approach minimizes the use of harmful chemicals and protects biodiversity. A study by the University of California, Davis, for instance, demonstrated a 20% reduction in pesticide use through AI-driven pest management in almond orchards. The resulting reduction in greenhouse gas emissions from pesticide production and application further contributes to a smaller carbon footprint.
Comparing AI-Driven and Traditional Farming Techniques
A comparison reveals a clear advantage for AI-driven farming techniques in terms of environmental sustainability. Traditional methods often rely on blanket approaches to irrigation, fertilization, and pest control, leading to significant resource waste and environmental pollution. AI, on the other hand, enables precision and optimization, leading to a reduction in water consumption, fertilizer use, and pesticide application. This translates to lower greenhouse gas emissions, reduced soil degradation, and minimized water pollution. For example, a farmer using traditional methods might irrigate an entire field, even if only a portion needs water, leading to significant water waste. An AI-powered system, however, would only irrigate the areas requiring water, conserving a significant amount of this precious resource.
A Plan for Improving Food Access and Affordability in Underserved Communities
AI can play a crucial role in improving food access and affordability in underserved communities by optimizing food distribution networks, predicting demand, and reducing food waste. This involves developing AI-powered logistics systems that optimize transportation routes, minimizing spoilage and ensuring timely delivery of food to remote areas. AI can also be used to predict food demand in specific regions, enabling more efficient food production and distribution to match local needs. Furthermore, AI-powered systems can help to identify and address food insecurity hotspots, allowing for targeted interventions and resource allocation. An example of such an initiative could be a system that uses data from mobile phone usage, social media, and weather patterns to predict food shortages in vulnerable communities, enabling timely interventions by aid organizations. By optimizing logistics and reducing waste, AI can make food more affordable and accessible, contributing to food security in underserved communities.
Ethical Considerations of AI in Food Production
The integration of artificial intelligence into food production, while promising increased efficiency and yield, raises significant ethical concerns that demand careful consideration. From algorithmic bias impacting food distribution to questions of job displacement and environmental sustainability, the responsible development and deployment of AI in this sector is paramount. This section delves into these crucial ethical dimensions.
Impact on Food Security and Access
AI’s influence on global food security is multifaceted. While precision agriculture techniques driven by AI can boost crop yields and reduce waste, concerns remain regarding equitable access to these technologies. The high cost of implementing AI systems could exacerbate existing inequalities, potentially widening the gap between developed and developing nations. Furthermore, the concentration of AI-powered food production in the hands of a few large corporations might limit competition and negatively affect smaller farmers and consumers. For example, a scenario where AI-driven farms dominate the market could lead to price hikes for consumers in less-developed regions lacking access to these technologies. This could further intensify existing food insecurity challenges within vulnerable populations.
Transparency and Accountability in AI Food Systems
Ensuring transparency and accountability in the development and deployment of AI within the food industry is crucial for building public trust. This requires clear guidelines on data collection, algorithm design, and decision-making processes. Open-source algorithms and accessible data sets can promote scrutiny and encourage collaboration amongst researchers, developers, and stakeholders. Independent audits of AI systems used in food production can help identify and address potential biases or unintended consequences. Furthermore, robust regulatory frameworks are necessary to ensure compliance with ethical standards and to hold developers accountable for the impacts of their technologies. A lack of transparency, for example, could lead to the use of AI systems that perpetuate unfair practices, such as price manipulation or discriminatory allocation of resources.
Potential Biases in AI Algorithms and Mitigation Strategies
The potential for bias in AI algorithms used in food production is significant and needs careful attention. These biases can stem from flawed data sets, biased algorithms, or human biases embedded in the system’s design.
- Bias 1: Data Bias Reflecting Existing Inequalities: AI algorithms trained on historical data that reflects existing inequalities (e.g., land ownership patterns, access to resources) may perpetuate and amplify these inequalities. Mitigation: Employing diverse and representative datasets, actively seeking data from marginalized communities, and implementing fairness-aware algorithms are crucial steps. This includes incorporating data from smaller farms and diverse agricultural practices to create a more balanced representation of the food system.
- Bias 2: Algorithmic Bias Favoring Specific Crops or Regions: AI systems might prioritize certain crops or regions based on factors like profitability or ease of implementation, potentially neglecting the needs of diverse agricultural ecosystems and local food systems. Mitigation: Developing algorithms that consider ecological sustainability and social equity alongside economic efficiency is vital. This requires a multi-criteria decision-making approach that balances various factors and prioritizes long-term sustainability over short-term gains.
- Bias 3: Lack of Consideration for Cultural Preferences and Dietary Needs: AI-driven food production systems may fail to account for diverse cultural preferences and dietary needs, leading to homogenization of food production and potentially reducing access to traditional foods. Mitigation: Incorporating diverse culinary traditions and dietary requirements into the design and implementation of AI systems is essential. This requires engagement with diverse communities and a commitment to preserving culinary heritage and promoting food diversity.
Final Thoughts
Alphabet X’s foray into AI-driven food production holds immense potential for transforming our food system. From optimizing agricultural practices to enhancing food processing and ensuring sustainability, the implications are far-reaching. However, navigating the ethical considerations and ensuring equitable access to these advancements are crucial for realizing the full benefits. The future of food is being written now, and AI is playing a pivotal role.