Uber scaled back sensors self driving cars – Uber scaled back sensors self-driving cars – the news sent shockwaves through the autonomous vehicle industry. This move, impacting lidar, radar, and camera systems, raises serious questions about the future of self-driving technology. Was it a cost-cutting measure, a strategic shift, or a sign of unforeseen technological hurdles? The implications for safety, competition, and Uber’s overall autonomous vehicle strategy are profound, and the answers aren’t straightforward.
This decision by Uber to reduce sensor technology in its self-driving fleet isn’t just about cost-cutting; it’s a complex interplay of technological limitations, business strategies, and ethical considerations. We delve into the technical challenges, the impact on safety, and what this means for the future of autonomous vehicles. Buckle up, it’s a wild ride.
Uber’s Self-Driving Program Reduction
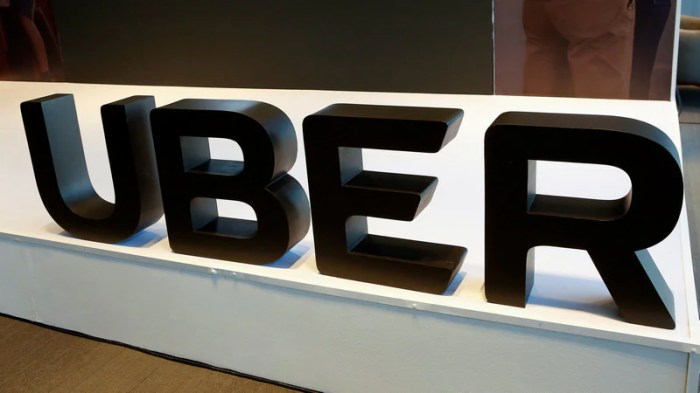
Source: gadgets360cdn.com
Uber’s ambitious foray into the autonomous vehicle market has seen its share of twists and turns, most recently a significant scaling back of its sensor technology. This strategic shift, while potentially cost-effective, raises crucial questions about the safety and efficacy of their self-driving cars. The move reflects a broader industry trend of reevaluating the optimal sensor suite for autonomous vehicles, a balance between comprehensive perception and economic viability.
Uber’s reduction in sensor technology represents a significant departure from their earlier, more sensor-laden approach. The company has opted to reduce its reliance on expensive and complex LiDAR systems, instead focusing on a more camera-centric approach supplemented by radar. This means fewer of the laser-based LiDAR units, which create detailed 3D maps of the environment, will be utilized. While cameras and radar remain crucial components, the reduced LiDAR integration signifies a shift towards a potentially less robust, yet more cost-effective, perception system.
Impact on Safety and Performance
The decreased sensor redundancy inherent in Uber’s revised approach undeniably impacts the safety and performance of its autonomous vehicles. LiDAR, with its high-resolution point cloud data, offers a superior level of detail compared to cameras and radar, especially in challenging conditions like low light or inclement weather. The reduction in LiDAR could lead to a higher reliance on computationally intensive image processing algorithms, potentially increasing the latency in object detection and decision-making. This increased reliance on camera-based vision might lead to difficulties in discerning objects accurately in adverse weather or lighting, increasing the risk of accidents. The trade-off is between the cost savings from fewer LiDAR units and a potential increase in the risk of failure. This strategy contrasts sharply with companies like Waymo, which heavily utilize LiDAR alongside other sensors for a highly redundant and robust perception system.
Comparison with Other Autonomous Vehicle Developers
Uber’s decision contrasts significantly with the strategies employed by other leading autonomous vehicle developers. Companies like Waymo, for example, are known for their highly redundant sensor suites, incorporating multiple LiDAR units, radar, and numerous cameras. This approach prioritizes safety and reliability, aiming for a robust perception system capable of handling a wide range of scenarios. Tesla, on the other hand, has famously opted for a camera-centric approach, albeit with a vastly different computational architecture and data processing capabilities than Uber’s current system. The differing approaches highlight the ongoing debate within the industry about the optimal balance between sensor redundancy, cost, and performance.
Timeline of Uber’s Self-Driving Program
The following table Artikels key milestones and setbacks in Uber’s self-driving program, showing the evolution of their sensor technology strategy:
Date | Event | Impact on Sensor Technology | Overall Program Impact |
---|---|---|---|
2015 | Uber acquires Otto, a self-driving truck startup. | Initial focus on LiDAR and other sensor fusion. | Expansion of self-driving initiatives. |
2016 | Launch of Uber ATG (Advanced Technologies Group). | Continued investment in diverse sensor technology. | Significant growth and expansion of R&D efforts. |
2018 | Fatal accident involving an Uber self-driving car. | Increased scrutiny of sensor technology and safety protocols. | Major setback, leading to temporary suspension of testing. |
2020 | Significant scaling back of self-driving efforts. | Reduction in LiDAR usage, shift towards camera-centric approach. | Reduced investment and focus on self-driving technology. |
2023 | Continued focus on limited deployment and strategic partnerships. | Ongoing refinement of sensor suite based on data and experience. | Program continues, but with a significantly reduced scope and ambition. |
Technological Reasons Behind the Reduction
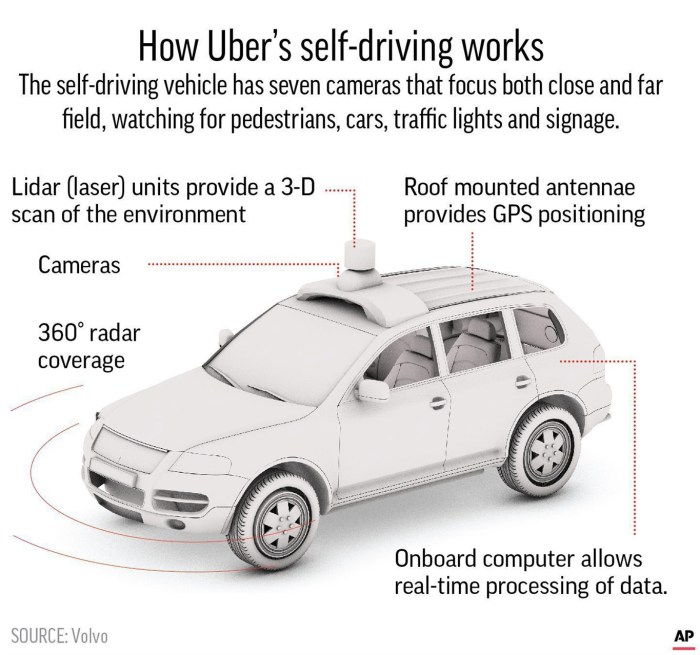
Source: mymcmurray.com
Uber’s scaling back on sensors for its self-driving cars highlights the complexities of autonomous vehicle tech. This shift in focus makes you wonder about the future of sophisticated sensor arrays, especially considering advancements like biometric security; check out this patent for Face ID coming to the Apple Watch, apple patent face id coming to apple watch , which shows how miniaturization and accuracy are pushing boundaries.
Ultimately, the lessons learned from Uber’s approach might influence how other companies approach sensor technology in autonomous systems.
Uber’s scaling back of sensors in its self-driving cars wasn’t a random decision; it was a calculated move driven by a complex interplay of technological hurdles, cost pressures, and a reassessment of the necessary level of redundancy for safe and efficient operation. The reduction reflects a shift in strategy, prioritizing a more streamlined and cost-effective approach, even if it means sacrificing some level of sensor-based redundancy.
The primary technological challenges that led to the sensor reduction are multifaceted. The sheer volume of data generated by a dense array of LiDAR, radar, and camera sensors was overwhelming. Processing this data in real-time, with the necessary accuracy and speed to make critical driving decisions, presented a significant computational bottleneck. This bottleneck impacted not only the performance of the self-driving system but also the overall cost and energy consumption.
Sensor Technology Costs and Integration
The cost of different sensor technologies varies drastically. LiDAR, while offering high-resolution 3D point cloud data, is significantly more expensive than radar or cameras. Integrating multiple sensor systems requires sophisticated hardware and software, adding further to the expense. For instance, the calibration and synchronization of different sensor modalities, ensuring that their data aligns perfectly, is a complex and time-consuming process. This integration complexity further amplifies the overall cost, especially when dealing with a large number of sensors. Uber likely found that the diminishing returns from adding more sensors did not justify the escalating costs. For example, the incremental improvement in accuracy obtained by adding a fifth LiDAR unit might be negligible compared to its cost and the increased computational load.
Sensor Redundancy, Accuracy, and Computational Complexity
The trade-off between sensor redundancy, accuracy, and computational complexity is central to the sensor reduction strategy. While multiple sensors provide redundancy, increasing robustness against sensor failure, they also increase the computational burden. Processing data from numerous sensors demands significantly more powerful processors and increased energy consumption. Uber likely determined that a certain level of redundancy could be sacrificed without compromising safety, particularly if advanced algorithms could compensate for the loss of some sensor data. The accuracy of the self-driving system is directly impacted by the quality and quantity of sensor data. However, Uber’s decision suggests that a reduction in sensor density, coupled with improved algorithms, could still maintain an acceptable level of accuracy.
Sensor Data Processing Algorithms and Software
The algorithms and software used to fuse data from multiple sensors are crucial for the performance of a self-driving system. These algorithms employ techniques like Kalman filtering and sensor fusion to integrate data from different sensor modalities, creating a consistent and accurate representation of the environment. Reducing the number of sensors necessitates a modification of these algorithms. Instead of relying on redundant data from multiple sensors, the system might rely more heavily on advanced data processing techniques to extract more information from fewer data sources. This might involve employing more sophisticated machine learning models that can learn to compensate for the reduced sensor input, making more accurate predictions about the environment based on available data. For instance, a model trained on a larger dataset might be able to infer the presence of an object even if it’s partially obscured from one sensor’s view.
Data Processing Pipeline Comparison
A flowchart illustrating the data processing pipeline would show a significant difference in complexity between the original system and the scaled-back version. The original pipeline would involve multiple parallel streams of data processing for each sensor type, followed by a complex fusion stage. The scaled-back version would have fewer input streams, simplifying the fusion process. However, the individual processing steps for each remaining sensor might become more computationally intensive as the system relies more heavily on advanced algorithms to compensate for the lack of redundant data. For example, in the original system, a missing data point from one LiDAR could be easily compensated for by data from another. In the scaled-back system, advanced algorithms would need to fill in this missing information based on contextual cues and predictions from other sensors. This necessitates more complex and computationally intensive algorithms.
Business and Strategic Implications
Uber’s decision to scale back on sensors for its self-driving cars carries significant business and strategic implications, potentially altering its trajectory in the autonomous vehicle race. While Uber hasn’t issued a formal, detailed public statement explicitly outlining the *why* behind the sensor reduction, we can infer their reasoning from the context of their overall self-driving program restructuring and the industry’s technological advancements. The move suggests a shift in their approach, prioritizing cost-effectiveness and potentially focusing on specific applications rather than a fully comprehensive, universally applicable autonomous system.
Uber’s official stance, gleaned from various press releases and statements by executives, emphasizes a refocus on efficiency and profitability within their autonomous vehicle division. The reduction in sensor reliance likely reflects a strategic bet on leveraging advanced software and algorithms to compensate for the reduced hardware, aiming for a more cost-effective solution. This approach contrasts with the earlier, more sensor-heavy approach favored by many competitors.
Uber’s Long-Term Autonomous Vehicle Development Strategy
The scaled-back sensor approach indicates a shift in Uber’s long-term autonomous vehicle strategy. The company may be moving away from a pursuit of fully autonomous vehicles capable of operating in all conditions towards a more phased rollout, focusing initially on applications with less demanding environmental complexity. This could mean prioritizing geofenced areas or specific operational scenarios, such as highway driving or deliveries in controlled environments. This approach mirrors the strategy of some competitors who are focusing on niche applications before tackling the broader challenges of full autonomy. The long-term success will depend on the ability of their software to reliably handle the complexities of real-world driving with fewer sensors. A failure to achieve this could significantly delay their progress and impact their overall market share.
Uber’s Competitive Position in the Autonomous Vehicle Market
The sensor reduction places Uber in a different competitive position. While companies like Waymo and Cruise continue to invest heavily in highly sensor-laden vehicles striving for Level 5 autonomy, Uber’s strategy represents a more cost-conscious approach. This could allow for faster deployment in certain applications, but also potentially limits the scope of their autonomous operations. The long-term success hinges on whether Uber can achieve comparable safety and reliability with fewer sensors, and whether their chosen approach proves to be more economically viable than the sensor-rich approaches of its competitors. The race is no longer solely about technological superiority, but also about efficient deployment and market penetration.
Implications for Uber’s Investments and Partnerships
The sensor reduction likely affects Uber’s investment strategy and partnerships. It may lead to a reassessment of collaborations with sensor manufacturers and a shift towards investing more heavily in software development and AI capabilities. Partnerships focusing on highly specialized sensors or mapping technologies might become less critical, while collaborations focused on software and data processing could become more important. The overall financial commitment to self-driving technology might be reduced, reflecting a more focused and potentially less risky approach. This could impact their ability to attract and retain top talent in the field.
Potential Short-Term and Long-Term Business Consequences
The shift to fewer sensors presents both opportunities and risks. A well-executed strategy could lead to significant cost savings and faster deployment of autonomous services in specific applications. However, there are potential downsides.
Here’s a breakdown of potential consequences:
- Short-Term Consequences: Reduced development costs, potentially faster deployment in limited environments, but also potential setbacks in achieving full autonomy in complex scenarios, and a potential impact on investor confidence.
- Long-Term Consequences: Increased profitability if the strategy proves successful, but also the risk of falling behind competitors in the race for full autonomy, limited market share in certain segments, and challenges in attracting and retaining top talent in the autonomous vehicle sector.
Safety and Ethical Considerations
Uber’s decision to scale back sensors on its self-driving cars raises significant safety and ethical concerns. Reducing sensor redundancy inherently increases the risk of accidents, impacting not only the safety of passengers and pedestrians but also the public’s perception and acceptance of autonomous vehicles. This section will explore the potential ramifications of this decision in various scenarios.
Impact of Sensor Reduction on Safety
The reduction in sensor capabilities directly impacts the self-driving car’s ability to perceive its environment accurately. Fewer sensors mean less data for the system to process, potentially leading to misinterpretations of the surroundings. For example, a reduction in lidar sensors could compromise the car’s ability to accurately judge distances, particularly in low-light conditions or when encountering unexpected obstacles like pedestrians or cyclists emerging from behind parked cars. Similarly, a reduction in camera resolution or the number of cameras might lead to difficulties in object recognition, especially with smaller or less distinct objects. This could lead to delayed or inaccurate braking or steering responses, increasing the likelihood of collisions. A reliance on fewer, potentially less robust, sensors increases the system’s vulnerability to sensor failure, which could have catastrophic consequences.
Ethical Implications of Reduced Sensor Systems
Deploying autonomous vehicles with fewer sensors creates significant ethical dilemmas, primarily concerning accident liability and public trust. If an accident occurs due to a sensor limitation, determining liability becomes complex. Is it the manufacturer’s fault for designing a less safe system, the software developer’s for inadequate algorithms, or the user’s for relying on a potentially deficient technology? The lack of redundancy and the potential for increased failure rates erode public trust in autonomous vehicles. A single high-profile accident caused by a sensor deficiency could significantly damage public confidence, hindering the broader adoption of self-driving technology. This lack of trust could also impact the insurance industry’s willingness to cover autonomous vehicles with reduced sensor capabilities, potentially making them less economically viable.
Comparative Safety Performance
Imagine two hypothetical scenarios. Scenario A involves a self-driving car equipped with a comprehensive sensor suite (multiple lidar, radar, and high-resolution cameras) navigating a busy city intersection. The car easily detects a pedestrian unexpectedly stepping into the street and smoothly brakes to avoid a collision. Scenario B involves a similar situation, but the car has a scaled-back sensor system. Due to limited data, the system misinterprets the pedestrian’s movement or fails to detect them entirely, resulting in a collision. This illustrates the stark difference in safety performance between autonomous vehicles with varying sensor configurations. The reliability and safety of the system are directly correlated to the quantity and quality of sensor data available.
Mitigation Strategies for Safety Concerns
Addressing the safety concerns arising from sensor reduction requires a multi-pronged approach. Firstly, advanced software algorithms are crucial. These algorithms must be designed to compensate for the limitations of a reduced sensor suite, employing sophisticated data fusion techniques to maximize the utility of the available data and minimize the risk of misinterpretations. Secondly, robust testing and validation are paramount. Rigorous simulations and real-world testing are needed to identify and address potential weaknesses in the system’s perception and decision-making capabilities. Thirdly, increased reliance on high-definition mapping and localization technologies could help compensate for reduced sensor inputs, providing additional context and information to the autonomous driving system. Finally, transparent communication with the public regarding the limitations of the scaled-back system is essential to build and maintain trust.
Hypothetical Accident Scenario
Consider a scenario where an Uber self-driving car with a scaled-back sensor system is approaching a dimly lit intersection. Due to a limited number of cameras and reduced lidar range, the car fails to detect a cyclist emerging from a side street at a low speed. The car’s decision-making system, relying on the insufficient sensory input, proceeds through the intersection, resulting in a collision with the cyclist. The consequences could range from minor injuries to severe trauma or even fatality for the cyclist, alongside significant legal and reputational damage for Uber. The investigation into the accident would likely focus on the inadequacy of the sensor system and the limitations of the associated algorithms in handling such low-visibility situations.
Future Directions for Uber’s Autonomous Vehicle Program: Uber Scaled Back Sensors Self Driving Cars
Uber’s scaled-back approach to self-driving technology doesn’t signal the end of the road; rather, it represents a strategic recalibration. By focusing resources, the company can potentially achieve a more robust and efficient autonomous driving system in the long run, prioritizing safety and practicality over sheer sensor density. This refocusing allows for a more targeted approach to technological advancements.
The shift necessitates a reassessment of the entire autonomous vehicle program, from sensor technology and software algorithms to the very definition of successful autonomous driving. This new direction will likely involve a leaner, more targeted approach, prioritizing cost-effectiveness and reliability over sheer technological ambition.
Uber’s Potential Future Sensor Technology Plans
The reduction in sensors doesn’t mean a complete abandonment of existing technology. Instead, Uber will likely refine its reliance on the remaining sensors – likely cameras and radar – by improving their capabilities and developing more sophisticated data processing techniques. This could involve exploring higher-resolution cameras with improved low-light performance and more advanced radar systems capable of distinguishing between objects with greater accuracy. The focus will be on maximizing the information extracted from fewer sources. For example, instead of relying on multiple lidar units for 360-degree perception, they might leverage advanced computer vision algorithms to interpret data from a smaller number of strategically placed cameras.
Software and Algorithm Adaptations for Reduced Sensor Input, Uber scaled back sensors self driving cars
With fewer sensors providing input, Uber’s software and algorithms will need to become significantly more intelligent and robust. This will involve developing more sophisticated fusion algorithms that can effectively combine data from different sensor modalities, such as cameras and radar, to create a comprehensive and accurate representation of the vehicle’s surroundings. Machine learning techniques will be crucial in training these algorithms to handle noisy or incomplete data, effectively compensating for the reduced sensor input. This might involve the development of novel deep learning models capable of filling in gaps in sensor data using contextual information and predictive modeling. Think of it as teaching the car to “see” more effectively with less information.
Research and Development Roadmap for Improved Performance with Fewer Sensors
A potential research and development roadmap could focus on three key areas: (1) Advanced Sensor Fusion: Developing algorithms that can seamlessly integrate data from diverse sensors (cameras, radar, potentially even ultrasonic sensors) to overcome limitations of individual sensors. (2) Robustness and Fault Tolerance: Designing systems that can reliably operate even with sensor failures or degraded data quality. This could involve redundant systems and sophisticated error correction techniques. (3) Contextual Understanding: Enhancing the system’s ability to utilize prior knowledge and environmental context to interpret ambiguous sensor data. This involves leveraging high-definition maps and real-time traffic data to anticipate potential hazards. This roadmap would prioritize incremental improvements, validating each stage through rigorous testing. For example, initial testing might focus on improved camera-radar fusion in controlled environments, followed by progressively more complex real-world scenarios.
Potential Alternative Sensor Technologies
While cameras and radar are likely to remain core components, Uber might explore supplementary technologies. For instance, high-resolution imaging sensors, possibly with spectral imaging capabilities, could provide richer information about the environment. Another area of interest could be improved sensor placement, optimizing sensor positioning to maximize coverage and minimize blind spots. The exploration of these alternative technologies would be iterative, with rigorous evaluation of their cost-effectiveness and performance benefits before widespread adoption.
The Role of Simulation and Virtual Testing
Simulation and virtual testing will be absolutely crucial in the development and validation of Uber’s future autonomous vehicle systems. The ability to create realistic virtual environments allows for extensive testing of algorithms and systems under a wide range of conditions, including edge cases that are difficult or impossible to replicate in the real world. This approach significantly reduces the need for costly and time-consuming real-world testing, accelerating the development process while ensuring safety. For example, simulations can replicate various weather conditions (rain, snow, fog), traffic scenarios, and road types to rigorously evaluate the robustness of the system. The integration of high-fidelity simulations into the development cycle will be key to achieving a safe and reliable autonomous driving system with a reduced sensor suite.
Closing Summary
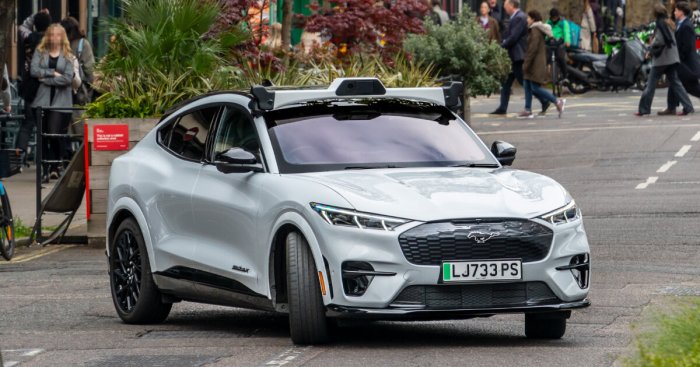
Source: designboom.com
Uber’s decision to scale back sensors on its self-driving cars marks a pivotal moment in the autonomous vehicle race. While cost and complexity played a role, the long-term implications for safety and public trust are undeniable. The move forces a critical re-evaluation of the technology’s readiness and the balance between ambition and practicality. Whether this signals a temporary setback or a fundamental shift in approach remains to be seen, but one thing is clear: the path to fully autonomous vehicles is far from smooth.