Advertisement
Ubers self driving program had problems before fatal crash – Uber’s self-driving program had problems before a fatal crash, raising serious questions about the safety and testing of autonomous vehicle technology. The accident highlighted not only technological shortcomings but also crucial ethical and regulatory concerns surrounding the rapid development and deployment of self-driving cars. This deep dive explores the incidents, near misses, and regulatory issues that preceded the tragic event, examining the role of human oversight, data analysis practices, and the inherent limitations of the technology itself. We’ll unpack the complexities of a system striving for autonomy while grappling with the unpredictable nature of real-world driving.
From software glitches and sensor limitations to the training and responsibilities of human safety drivers, we’ll analyze the contributing factors that potentially led to the fatal crash. We’ll also examine the public perception and the ethical dilemmas inherent in the development of this groundbreaking, yet potentially hazardous, technology. Get ready for a critical look at a pivotal moment in the history of autonomous vehicles.
Uber’s Self-Driving Technology Before the Fatal Crash
The fatal crash involving Uber’s self-driving vehicle in 2018 cast a long shadow over the autonomous vehicle industry. Understanding the technology’s capabilities and limitations before the accident is crucial to comprehending the event and its aftermath. This examination focuses on the technological underpinnings of Uber’s self-driving system, its safety features, and the testing procedures employed prior to the tragic incident.
Uber’s self-driving system relied on a complex interplay of hardware and software. The hardware included an array of sensors, such as lidar (light detection and ranging), radar, and cameras, providing a 360-degree view of the vehicle’s surroundings. This sensor data was then processed by powerful onboard computers running sophisticated algorithms designed to perceive objects, predict their movements, and make driving decisions. The system’s software incorporated machine learning techniques, constantly learning and improving its performance through the analysis of vast amounts of driving data. A crucial element was the “safety driver,” a human operator tasked with monitoring the system and intervening if necessary.
Safety Features and Their Intended Functions
The system incorporated several safety features intended to mitigate risks. These included automatic emergency braking, lane keeping assist, and adaptive cruise control. Automatic emergency braking was designed to automatically apply the brakes if the system detected an imminent collision. Lane keeping assist was meant to prevent the vehicle from drifting out of its lane, while adaptive cruise control maintained a safe following distance from other vehicles. The safety driver’s role was paramount, acting as a final safety net to override the autonomous system if necessary. The intention was for these features to work in concert, creating multiple layers of redundancy to prevent accidents.
Testing Methodologies Employed by Uber
Uber employed a multi-phased testing methodology for its self-driving vehicles. These phases involved progressively more complex driving scenarios and environments. The initial phases focused on controlled environments, gradually increasing in complexity until testing in real-world traffic conditions. The following table summarizes the different phases and their outcomes:
Phase | Location | Duration | Notable Incidents |
---|---|---|---|
Simulated Environment Testing | Computer Simulations | Ongoing | Various software bugs and algorithm failures identified and addressed. |
Closed-Course Testing | Private Test Tracks | Several Months | Minor incidents involving software glitches and sensor malfunctions; no serious accidents. |
Limited Public Road Testing | Selected Urban Areas | Several Months | Several near misses reported, leading to adjustments in software and sensor configurations. |
Expanded Public Road Testing | Multiple Cities | Several Months | Increased frequency of near misses; the fatal accident occurred during this phase. |
Reported Incidents and Near Misses Leading Up to the Crash
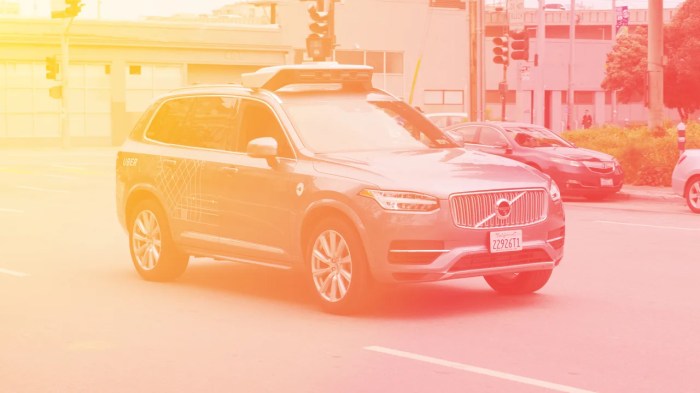
Source: fastcompany.net
The fatal accident involving Uber’s self-driving car in Tempe, Arizona, wasn’t an isolated incident. A review of internal documents and investigations revealed a series of prior near-misses and incidents involving the autonomous vehicles, highlighting concerns about the system’s safety and the company’s response to these warning signs. These events, ranging from minor glitches to more serious near-collisions, paint a picture of a technology struggling to reliably navigate complex real-world driving scenarios. Understanding these preceding incidents is crucial for analyzing the root causes of the fatal crash.
The incidents weren’t always publicly disclosed, adding to the complexity of evaluating Uber’s handling of safety concerns. Internal reports, however, detail a range of issues that ultimately contributed to a culture that may have downplayed the severity of these near misses. Analyzing these incidents chronologically provides valuable insights into the evolution of the problems and the company’s evolving response.
Incidents Involving Unexpected Braking and Acceleration
Several incidents involved unexpected braking or acceleration by Uber’s self-driving vehicles. One report detailed a situation where a vehicle unexpectedly braked hard while approaching an intersection, causing a following car to nearly rear-end it. Another involved an instance of unintended acceleration, resulting in a near-collision with a pedestrian. These incidents highlight potential flaws in the vehicle’s control systems and the need for more robust fail-safes. The contributing factors often involved misinterpretations of sensor data, leading to erratic vehicle behavior. Uber’s response to these incidents varied; some resulted in software updates, while others seemingly led to minimal internal investigation.
Near Misses with Pedestrians and Cyclists
A significant number of near-misses involved vulnerable road users like pedestrians and cyclists. One incident involved a self-driving car narrowly avoiding a collision with a pedestrian crossing the street outside of a crosswalk. Another saw a cyclist nearly hit after the autonomous vehicle failed to correctly interpret the cyclist’s trajectory. These incidents underscored the challenges of accurately perceiving and responding to unpredictable movements of pedestrians and cyclists, particularly in complex urban environments. The company’s internal responses to these incidents, according to reports, often focused on refining the perception algorithms but lacked a comprehensive review of the overall safety protocols.
Incidents Related to Object Recognition and Lane Keeping, Ubers self driving program had problems before fatal crash
Problems with object recognition and lane keeping also contributed to several near-misses. In one instance, a self-driving car failed to correctly identify a stopped vehicle in its lane, leading to a near-collision. Another involved the vehicle drifting out of its lane, necessitating manual intervention by the safety driver. These incidents highlighted shortcomings in the system’s ability to reliably perceive its surroundings and maintain its position on the road. The responses to these incidents often focused on improving the vehicle’s sensor fusion algorithms and refining the lane-keeping capabilities. However, a consistent and proactive approach to safety across all incidents was reportedly lacking.
Regulatory Oversight and Compliance Issues
The fatal crash involving Uber’s self-driving car highlighted significant gaps in the regulatory landscape surrounding autonomous vehicle testing and the company’s adherence to existing rules. The lack of clear, comprehensive, and consistently enforced regulations created a grey area that allowed Uber to operate with potentially insufficient oversight, ultimately contributing to the tragic outcome. This section examines the regulatory framework in place at the time and Uber’s relationship with regulatory bodies.
The regulatory environment for autonomous vehicles in 2018, when the crash occurred, was still nascent and fragmented. Different states and municipalities had varying regulations, creating a patchwork of rules that lacked national consistency. While some jurisdictions had established permitting processes and testing guidelines, others had minimal or no specific regulations for self-driving car programs. This lack of uniform standards made it difficult to establish clear benchmarks for safety and operational compliance. Furthermore, the rapid technological advancements in the field outpaced the development of appropriate legal frameworks, leaving significant regulatory gaps.
Arizona’s Regulatory Framework and Uber’s Operations
Arizona, where the fatal crash took place, had a relatively permissive regulatory environment for autonomous vehicle testing at the time. The state’s regulations focused primarily on permitting and reporting requirements, rather than detailed technical specifications or stringent safety protocols. Uber obtained a permit to test its self-driving vehicles in Arizona, but the specifics of that permit and the level of scrutiny applied during the testing phase remain subject to ongoing debate and scrutiny following the accident. While the permit allowed testing, it did not necessarily equate to comprehensive oversight or guarantee adherence to best practices for safety and operational protocols. The subsequent investigations revealed inconsistencies between Uber’s internal safety procedures and the standards expected by the regulatory authorities, highlighting a potential disconnect between self-reported compliance and actual practices.
Interactions Between Uber and Regulatory Bodies
The interactions between Uber and regulatory bodies, both before and after the crash, reveal a complex picture. While Uber obtained necessary permits to operate in Arizona, evidence suggests that communication and collaboration with regulatory agencies may not have been as robust or transparent as needed. The investigation following the accident uncovered internal communications indicating a potential downplaying of safety concerns within Uber, suggesting a lack of proactive engagement with regulators to address emerging issues. The ensuing regulatory scrutiny and subsequent fines levied against Uber underscored the importance of clear communication, proactive safety reporting, and a culture of compliance within autonomous vehicle programs. The lack of these elements ultimately contributed to the regulatory issues surrounding the incident.
The Role of Human Oversight and Driver Responsibilities: Ubers Self Driving Program Had Problems Before Fatal Crash
The Uber self-driving program relied heavily on a human safety driver, a crucial element intended to mitigate the risks associated with autonomous vehicle technology. Their presence was meant to provide a fail-safe mechanism, intervening when the system malfunctioned or encountered unexpected situations. However, the effectiveness of this oversight and the training provided to these drivers became a critical point of discussion following the fatal crash.
The human safety driver in Uber’s self-driving vehicles was tasked with monitoring the vehicle’s performance, taking control when necessary, and ensuring passenger safety. This included actively observing the vehicle’s behavior, the surrounding environment, and reacting appropriately to any potential hazards. Their responsibilities extended beyond simply being present; they were expected to be vigilant and ready to assume control at a moment’s notice.
Safety Driver Training and Experience
Uber’s safety drivers underwent a training program designed to familiarize them with the self-driving system and prepare them for various scenarios. The specifics of this training have been debated, with questions raised about its adequacy in preparing drivers for complex and unpredictable situations. The level of experience required also varied, and the balance between technological understanding and driving expertise was a key factor in evaluating their preparedness. Reports suggested that some drivers felt inadequately trained to handle the complexities of autonomous driving technology, particularly in unexpected circumstances.
Examples of Human Intervention and Accident Prevention
The following table illustrates scenarios where human intervention was required or could have potentially prevented accidents. It highlights the interplay between the autonomous system and the human driver, demonstrating the crucial role of human oversight.
Situation | Driver Action | System Response | Outcome |
---|---|---|---|
Unexpected pedestrian crossing unexpectedly in low-light conditions. | Driver took immediate control, braking to avoid collision. | System initially failed to detect the pedestrian. | Accident avoided. |
Sudden lane change by another vehicle, resulting in a near-miss. | Driver maintained vigilance and was prepared to intervene, but autonomous system successfully navigated the situation. | System detected the other vehicle and initiated corrective action. | Near-miss avoided. |
Construction zone with unexpected obstacles not mapped in the system. | Driver manually steered the vehicle around the obstacles. | System hesitated and showed uncertainty. | Accident avoided. |
Vehicle malfunction resulting in erratic driving behavior. | Driver immediately took control, bringing the vehicle to a safe stop. | System experienced a software glitch leading to unpredictable steering. | Accident avoided. |
Data Collection and Analysis Practices
Uber’s self-driving program relied heavily on data—a massive influx of information from sensors, cameras, and other onboard systems. This data was the lifeblood of the system’s development, fueling improvements in both performance and safety. The company’s approach, however, wasn’t without its complexities and potential pitfalls.
The data collection process involved a sophisticated array of sensors including lidar, radar, cameras, and GPS. These systems continuously gathered information about the vehicle’s surroundings – everything from the position and speed of other vehicles and pedestrians to the geometry of the road and the presence of traffic signals. This raw data was then transmitted to Uber’s servers for processing and analysis. Sophisticated algorithms were employed to filter, clean, and structure the data, preparing it for use in machine learning models.
Data Usage for System Improvement
This processed data served as the foundation for improving the self-driving system. Machine learning algorithms used this data to train the system’s perception, decision-making, and control modules. For example, by analyzing thousands of instances of vehicles approaching intersections, the system could learn to better predict the behavior of other drivers and make safer decisions about merging or stopping. Similarly, data from near-miss incidents allowed engineers to identify weaknesses in the system’s perception or decision-making capabilities and implement corrective measures. The iterative process of data collection, analysis, and system refinement was central to Uber’s approach.
Limitations and Biases in Data Collection
Despite the scale and sophistication of Uber’s data collection, inherent limitations and biases existed. One significant limitation stemmed from the geographical distribution of the data. The majority of testing occurred in specific areas, potentially leading to a system that performed well in those environments but poorly in others. This raises concerns about the generalizability of the system’s performance. Another crucial point is the potential for bias in the data itself. For instance, if the training data primarily reflected driving conditions in sunny, well-lit areas, the system might perform poorly in challenging conditions such as heavy rain or fog. This highlights the importance of ensuring diverse and representative data sets in the training process. Furthermore, the sheer volume of data could pose challenges in terms of storage, processing power, and the ability to effectively identify and address anomalies or edge cases within the data.
Software and Hardware Limitations
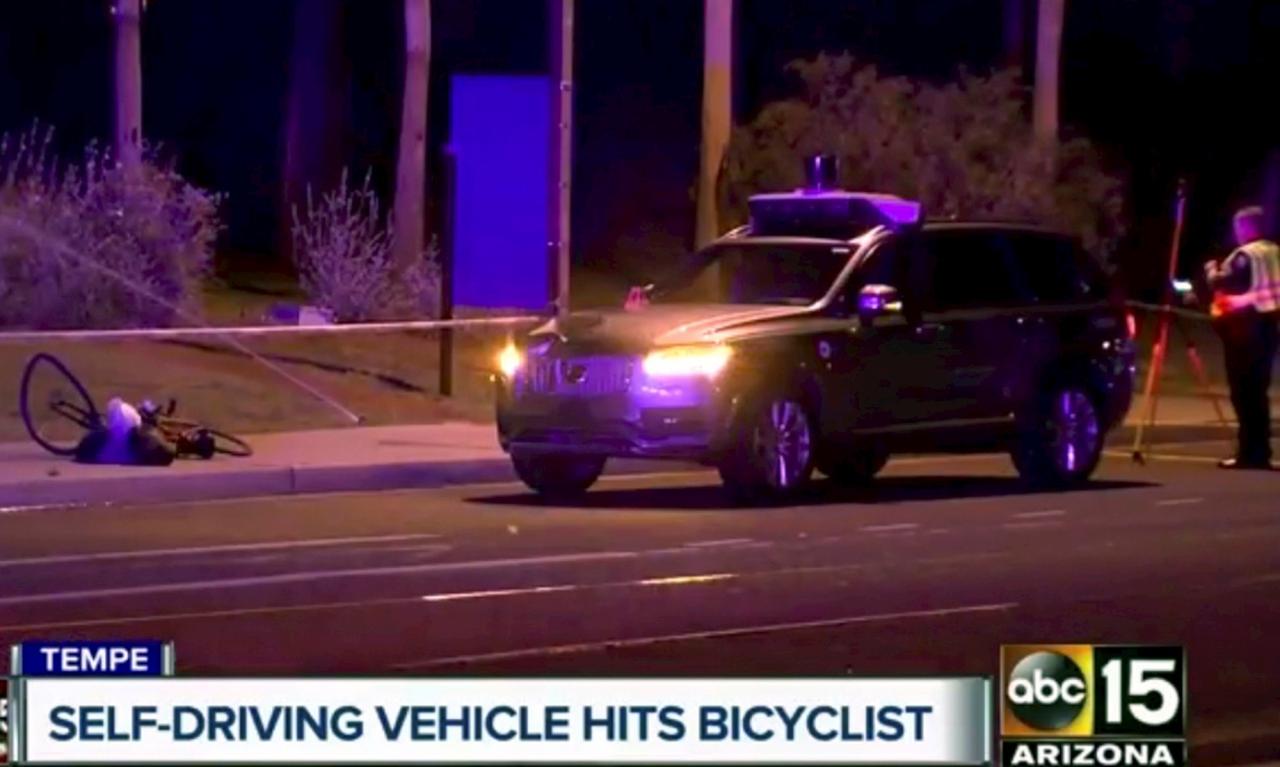
Source: co.nz
Uber’s self-driving program, plagued with glitches before its fatal accident, highlights the dangers of rushing untested tech. It’s a bit like trying to access apps from a different country’s Play Store, as detailed in this article: some android users can shop different country play store – you might find some cool stuff, but also some seriously buggy stuff that could cause unexpected problems.
Ultimately, both situations underscore the need for thorough testing before widespread deployment.
Uber’s self-driving system, while ambitious, relied on a complex interplay of software and hardware components. The fatal crash, and preceding incidents, highlighted critical vulnerabilities within this system, suggesting that the technology, at the time, was not robust enough to handle the unpredictable nature of real-world driving scenarios. Understanding these limitations is crucial to evaluating the safety and efficacy of autonomous vehicle technology.
The interplay between software and hardware created a cascading effect where limitations in one area exacerbated weaknesses in others. This wasn’t simply a matter of isolated failures, but rather a complex web of interconnected issues that ultimately led to the tragic outcome.
Sensor Limitations
Sensor data forms the bedrock of any autonomous driving system. Uber’s system, like many others, relied heavily on lidar, radar, and cameras to perceive its surroundings. However, these sensors have inherent limitations. For example, lidar struggles in adverse weather conditions like heavy rain or fog, significantly reducing its effective range and accuracy. Radar can be confused by objects with similar reflective properties, potentially misinterpreting a pedestrian for a roadside sign. Cameras, meanwhile, can be affected by glare, shadows, and low-light conditions, impacting object detection and recognition. These limitations could have individually or cumulatively contributed to the system misinterpreting the environment, leading to a failure to react appropriately to the presence of the pedestrian.
Software Bugs and Algorithm Limitations
The software controlling the self-driving system is incredibly complex, comprising millions of lines of code. This complexity inherently increases the risk of bugs, particularly in edge cases or unexpected situations. One potential limitation was the system’s inability to reliably process and interpret ambiguous or conflicting sensor data. For instance, if a lidar reading conflicted with a camera image, the software might have prioritized one data source over another, leading to an inaccurate assessment of the situation. Additionally, the algorithms used for decision-making might not have been sufficiently robust to handle the rapid and unpredictable changes that can occur in real-world driving scenarios, like sudden pedestrian movements or unexpected vehicle maneuvers.
Processing Power and Computational Constraints
Real-time processing of vast amounts of sensor data is crucial for safe autonomous driving. The computational power required to analyze this data, make decisions, and control the vehicle’s actions is substantial. If the system’s processing power was insufficient, it might have struggled to keep up with the demands of real-time operation, resulting in delayed responses or inaccurate calculations. This could have contributed to the system’s failure to adequately react to the pedestrian in the fatal crash, or to other near-miss incidents where rapid decision-making was critical. Furthermore, limitations in processing speed might have prevented the system from effectively integrating and prioritizing information from multiple sensors, leading to inaccurate perception and flawed decision-making.
Ethical Considerations and Public Perception
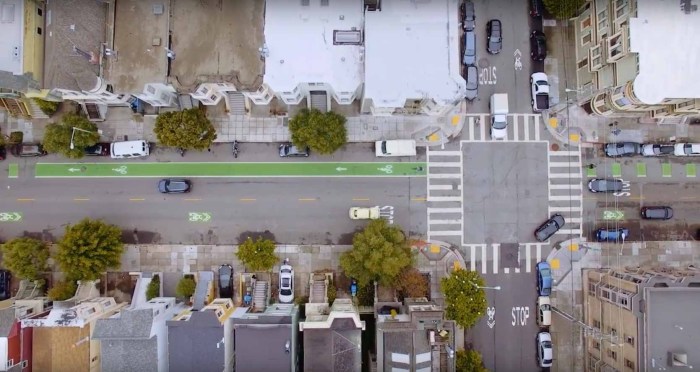
Source: sfbike.org
The tragic Uber self-driving car accident raised profound ethical questions about the development and deployment of autonomous vehicles, forcing a critical examination of the technology’s societal impact and the responsibilities of companies pushing its boundaries. The incident also dramatically shifted public perception, highlighting the complex interplay between technological advancement, safety concerns, and public trust.
The ethical dilemmas surrounding self-driving cars are multifaceted. One key issue is the programming of moral algorithms – how should a car be programmed to react in unavoidable accident scenarios? Should it prioritize the safety of its passengers over pedestrians? The lack of clear answers to these questions creates a moral grey area that companies like Uber must navigate carefully. Beyond the algorithmic level, there are broader ethical considerations regarding data privacy, job displacement due to automation, and the potential for biased algorithms to perpetuate existing societal inequalities. For example, if a self-driving car’s sensors are less accurate in identifying pedestrians with darker skin tones, the ethical implications are severe and unacceptable.
Public Perception Before and After the Fatal Crash
Before the fatal crash, public perception of Uber’s self-driving program was a mixture of excitement and apprehension. The promise of safer, more efficient transportation was alluring, but concerns about the technology’s readiness and potential risks were also voiced. Many saw it as a groundbreaking technological leap with the potential to revolutionize the transportation industry. However, this positive outlook was significantly impacted by the accident. News coverage extensively detailed the crash, leading to widespread skepticism and fear regarding the safety of autonomous vehicles. Public trust in Uber’s self-driving program plummeted, and the company faced intense scrutiny from regulators, the media, and the public. The accident served as a stark reminder of the potential consequences of deploying untested technology on public roads.
Impact on Public Trust in Autonomous Vehicle Technology
The Uber crash had a significant and lasting impact on public trust in autonomous vehicle technology as a whole. The incident fueled public anxieties about the reliability and safety of self-driving cars, raising doubts about whether the technology is sufficiently mature for widespread deployment. This erosion of trust was not limited to Uber; it affected the entire autonomous vehicle sector, potentially slowing down the adoption of this transformative technology. The accident highlighted the critical need for robust safety protocols, rigorous testing, and transparent communication from companies developing and deploying self-driving technology to regain public confidence. The long-term consequences of this loss of trust remain to be seen, but it undoubtedly presents a significant challenge for the industry’s future.
Last Point
The fatal crash involving Uber’s self-driving car wasn’t an isolated incident; it was the culmination of a series of near misses and underlying issues that exposed vulnerabilities in the company’s testing procedures, regulatory compliance, and the technology itself. The incident serves as a stark reminder of the high stakes involved in the development of autonomous vehicles and the critical need for robust safety protocols, transparent data analysis, and rigorous regulatory oversight. The quest for self-driving cars continues, but the path forward requires a renewed commitment to safety and ethical considerations, ensuring that the pursuit of innovation doesn’t come at the cost of human lives.