Advertisement
Google Deep Learning Model Pixel 2: Remember those mind-blowing Pixel 2 photos? Yeah, the ones that made other phones look… well, kinda sad. That magic wasn’t just good hardware; it was a deep learning revolution crammed into your pocket. This deep dive explores the AI wizardry behind the Pixel 2’s camera, from the models powering its HDR+ to the algorithms making low-light shots surprisingly brilliant. Get ready to peek behind the curtain of computational photography.
We’ll unpack the specific deep learning models used, how they were trained, and the impact on features like Super Res Zoom and Portrait Mode. We’ll even look at the limitations and future possibilities of this groundbreaking tech. Think of it as a backstage pass to the Pixel 2’s photographic prowess.
Google Pixel 2 Camera Capabilities
The Google Pixel 2, released in 2017, wasn’t just another smartphone; it was a camera phone revolution. While boasting relatively modest hardware compared to its competitors, it punched far above its weight thanks to Google’s masterful computational photography prowess. This wasn’t just about megapixels; it was about intelligent image processing that transformed snapshots into stunning visuals.
Image Processing Pipeline of the Google Pixel 2 Camera
The Pixel 2’s camera magic wasn’t solely in the hardware. Its image processing pipeline was a carefully orchestrated symphony of algorithms. First, the sensor captured raw image data. This raw data then underwent a series of sophisticated processes: noise reduction, HDR+ (High Dynamic Range Plus) processing for improved detail in both shadows and highlights, Super Res Zoom for clearer zoomed-in images, and finally, image sharpening and color correction to produce the final output. Google’s HDR+ was particularly groundbreaking, merging multiple exposures to create images with exceptional dynamic range and detail that far surpassed the capabilities of single-exposure shots. This multi-frame processing allowed the Pixel 2 to capture scenes with a much wider range of brightness levels than was previously possible on a smartphone.
Hardware Components Contributing to Image Quality
While software played a starring role, the Pixel 2’s hardware provided a solid foundation. It featured a 12.2-megapixel rear-facing camera with a relatively large sensor size (1/2.3″) for a smartphone at the time. This larger sensor allowed for better light gathering, crucial for low-light photography. The single-lens setup, while seemingly simple compared to multi-lens systems emerging in later phones, was a testament to the power of computational photography in compensating for hardware limitations. The aperture of f/1.8 allowed for a good balance of depth of field and light gathering. The absence of optical image stabilization (OIS) was largely mitigated by Google’s software-based image stabilization.
Computational Photography Techniques Enhancing Image Results
The Pixel 2’s success was largely attributed to its sophisticated computational photography techniques. HDR+ was a cornerstone, creating images with incredible dynamic range and detail. Super Res Zoom used multiple images to create a higher-resolution zoomed-in image, significantly reducing the typical artifacts associated with digital zoom. The Pixel Visual Core, a dedicated image processing chip, further accelerated these computationally intensive processes, ensuring near real-time performance. These algorithms weren’t just about technical improvements; they were about understanding how the human eye perceives images and replicating that in a digital format.
Comparison of Pixel 2 Camera Performance to Contemporary Smartphones, Google deep learning model pixel 2
At the time of its release, the Pixel 2’s camera consistently outperformed many contemporary smartphones, even those with higher megapixel counts. Reviewers frequently praised its superior dynamic range, detail, and low-light performance. While phones like the iPhone 8 boasted impressive camera systems, the Pixel 2 often edged out the competition in terms of image quality, particularly in challenging lighting conditions. This wasn’t a matter of simply having more megapixels; it was a demonstration of how smart software could surpass raw hardware capabilities.
Comparison of Pixel 2 Camera Specs to its Predecessor and Successor
Feature | Pixel | Pixel 2 | Pixel 3 |
---|---|---|---|
Main Camera Resolution | 12.3 MP | 12.2 MP | 12.2 MP |
Aperture | f/2.0 | f/1.8 | f/1.8 |
Optical Image Stabilization (OIS) | No | No | Yes |
Key Computational Features | HDR+ | HDR+, Super Res Zoom | HDR+, Super Res Zoom, Night Sight |
Deep Learning in Google Pixel 2 Image Processing
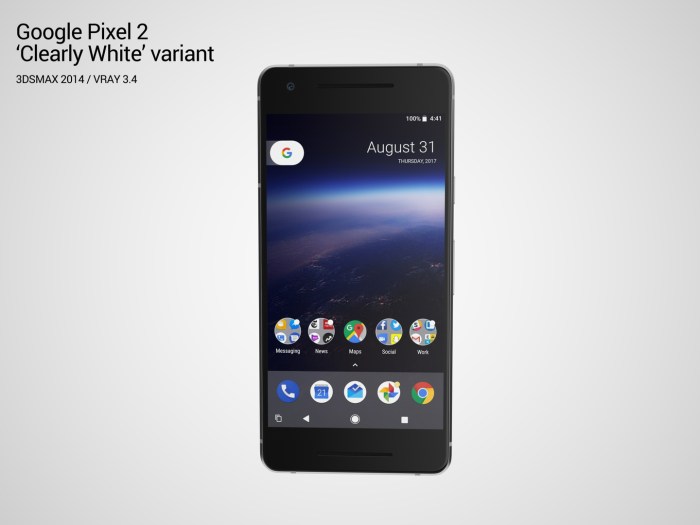
Source: turbosquid.com
The Google Pixel 2, despite its relatively modest hardware compared to competitors, delivered stunning image quality thanks to its clever use of deep learning. Instead of relying solely on powerful sensors and lenses, Google leveraged sophisticated algorithms to enhance various aspects of image processing, resulting in photos that punched above their weight class. This wasn’t just about simple filters; it was about fundamentally improving the core aspects of photography.
The Pixel 2’s camera software incorporated several deep learning models working in concert to achieve its impressive results. These models weren’t just simple image filters; they were complex neural networks trained on massive datasets of images, learning to identify and correct various imperfections and enhance various visual characteristics. This approach allowed for a level of image manipulation previously unseen in mobile photography.
Noise Reduction in the Pixel 2
The Pixel 2’s noise reduction capabilities were significantly enhanced by a deep learning model specifically trained to distinguish between actual image detail and noise artifacts. Traditional noise reduction methods often blurred fine details along with the noise, resulting in a loss of sharpness. The Pixel 2’s deep learning model, however, learned to selectively remove noise while preserving fine details like textures in clothing or the subtle features of a landscape. This resulted in cleaner images with significantly less grain, especially in low-light situations, without sacrificing image sharpness. For instance, a photo of a dimly lit street scene would retain the texture of the brickwork and the details of street signs, while significantly reducing the noise that would typically plague such an image.
HDR+ Enhancement via Deep Learning
Google’s HDR+ technology, a cornerstone of the Pixel 2’s imaging prowess, also benefited greatly from deep learning. The system takes multiple photos at different exposures and uses a deep learning model to fuse them together, creating an image with a significantly wider dynamic range than a single exposure could capture. This model learned to align the images perfectly, accounting for subtle movements and variations in lighting, resulting in images with more detail in both the highlights and shadows. A picture of a sunset, for example, would show both the vibrant colors of the sky and the fine details in the foreground without either being overexposed or underexposed.
Deep Learning’s Impact on Image Sharpness and Detail
Beyond noise reduction and HDR+, deep learning models in the Pixel 2 also played a significant role in enhancing image sharpness and detail. These models learned to identify and sharpen edges and fine details within an image, going beyond simple sharpening filters that often lead to artificial-looking halos around objects. The Pixel 2’s deep learning approach produced images with a more natural and refined sharpness, bringing out subtle textures and details that would otherwise be lost. Consider a portrait; the deep learning model would sharpen the eyes and the strands of hair, creating a more lifelike and detailed image without the harshness of traditional sharpening techniques.
Low-Light Photography Improvements
The Pixel 2’s low-light performance was a significant leap forward, largely due to the deep learning models employed. These models were trained on a vast dataset of low-light images, learning to effectively reduce noise and enhance detail even in challenging lighting conditions. The result was significantly improved image quality in low-light situations, with less noise and more detail than previous generations of mobile phone cameras could achieve. For example, a photo taken in a dimly lit restaurant would show significantly clearer details of faces and table settings compared to similar photos taken with other phones of the time.
Benefits of Deep Learning in Pixel 2 Camera
The benefits of incorporating deep learning into the Pixel 2’s camera system are numerous and significant. These models allowed for a substantial improvement in overall image quality, surpassing what was achievable with traditional image processing techniques.
- Improved noise reduction without sacrificing detail.
- Enhanced HDR+ capabilities for greater dynamic range.
- Increased image sharpness and detail with a natural look.
- Significantly better low-light performance.
- Overall improved image quality and consistency across various shooting conditions.
Model Architecture and Training
The Google Pixel 2’s impressive camera capabilities are heavily reliant on sophisticated deep learning models. These models aren’t simple; they’re complex networks trained on massive datasets to perform tasks like noise reduction, HDR+ image fusion, and super-resolution. Understanding their architecture and training process reveals the magic behind the Pixel 2’s photographic prowess.
The Pixel 2 likely employs a convolutional neural network (CNN) architecture, a type of deep learning model particularly well-suited for image processing. CNNs excel at extracting features from images thanks to their convolutional layers, which use filters to detect patterns at different scales. These extracted features are then processed through fully connected layers to produce the final output, which might be a denoised image, a merged HDR image, or a higher-resolution version of the original. The specific architecture would likely be a variation of well-known models, possibly incorporating residual connections (like ResNet) for improved training and performance, or incorporating attention mechanisms for focusing on relevant image regions.
Model Architecture Details
A likely architecture would involve multiple convolutional layers, each followed by activation functions (like ReLU) to introduce non-linearity. Pooling layers would reduce the spatial dimensions of the feature maps, decreasing computational cost and helping the model to be less sensitive to small variations in the input. The final layers would consist of fully connected layers that map the extracted features to the desired output, such as a pixel value for each channel (RGB). The output layer might also include a sigmoid or softmax function depending on the specific task. For instance, in a binary classification task (e.g., identifying whether an image contains a cat), a sigmoid function would output a probability between 0 and 1.
Training Data
Training such a model requires a massive dataset of images, ideally covering a wide range of lighting conditions, scenes, and object types. Google likely leveraged its vast resources to compile a dataset containing millions of images, possibly including images captured with various camera sensors, and images manually annotated for tasks like noise level or object location. This diverse dataset is crucial for ensuring the model generalizes well to unseen images. The data likely also includes paired images; for example, a noisy image paired with its clean counterpart for noise reduction training, or multiple exposures paired for HDR+ training.
Training Process
The training process involves feeding the model batches of images from the training dataset and calculating the difference between the model’s output and the ground truth (the desired output). This difference is quantified using a loss function, which measures the error. The model’s parameters (weights and biases) are then adjusted using an optimization algorithm, such as stochastic gradient descent (SGD) or Adam, to minimize the loss function. Hyperparameters, such as learning rate, batch size, and the number of training epochs, are carefully tuned to optimize the model’s performance. Regularization techniques, like dropout or weight decay, might be employed to prevent overfitting.
Comparison of Model Architectures
Several architectures could have been considered. While CNNs are the most likely choice, other architectures like recurrent neural networks (RNNs) or transformers could be incorporated for specific tasks. RNNs might be useful for sequential processing of image data, while transformers could excel at capturing long-range dependencies within an image. The final choice would depend on the specific task and a trade-off between accuracy, computational cost, and training time. For example, a simpler model might be sufficient for noise reduction, while a more complex model might be needed for tasks like super-resolution.
Simplified Neural Network for Noise Reduction
A simplified neural network for noise reduction could consist of three convolutional layers, each followed by a ReLU activation function and a max pooling layer. The first convolutional layer could use a small kernel size (e.g., 3×3) to detect local patterns, while subsequent layers could use larger kernels to capture more global features. The final layer would be a convolutional layer with the same number of channels as the input image, producing a denoised image. The loss function could be the mean squared error (MSE) between the denoised image and the ground truth clean image. The optimization algorithm could be Adam. This simplified model wouldn’t match the complexity of the Pixel 2’s actual model, but it illustrates the basic principles involved.
Impact of Deep Learning on Pixel 2 Features
The Google Pixel 2’s camera wasn’t just a hardware upgrade; it was a leap forward powered by the magic of deep learning. This sophisticated AI allowed the Pixel 2 to achieve photographic results previously unattainable in smartphones, transforming everyday snapshots into stunning images and videos. Let’s dive into how deep learning specifically boosted its key features.
Super Res Zoom Enhancement
Deep learning was instrumental in making Super Res Zoom on the Pixel 2 possible. Traditional digital zoom simply enlarges pixels, leading to blurry and pixelated images. However, the Pixel 2’s deep learning model analyzed multiple frames captured at lower resolutions, intelligently upscaling the image while maintaining detail and sharpness. This process involved a complex neural network trained on vast datasets of images, learning to predict high-resolution details from lower-resolution inputs. The result was a significantly improved zoom experience, delivering clearer images at higher zoom levels than competing phones could manage without significant quality loss. Think of it as the AI magically filling in the missing pixels with educated guesses based on its training.
Bokeh Effect in Portrait Mode
The Pixel 2’s impressive Portrait Mode, capable of producing professional-looking photos with blurred backgrounds (bokeh), heavily relied on deep learning. Instead of relying solely on hardware like dual lenses, the Pixel 2 used a single lens and a deep learning model to accurately segment the subject from the background. This model learned to distinguish between edges, textures, and lighting conditions to create a natural-looking depth-of-field effect. The AI precisely identified the subject’s boundaries, blurring the background while keeping the subject sharply in focus, even with complex hair or clothing. This precise segmentation was a direct result of the model’s training on millions of images, allowing it to handle diverse scenarios with remarkable accuracy.
Video Stabilization Improvements
Deep learning also played a crucial role in the Pixel 2’s video stabilization. Traditional image stabilization techniques often struggle with significant camera shake or movement. The Pixel 2, however, used a deep learning model to analyze video frames and predict future motion. This allowed for smoother, more stable videos even when recording while walking or moving. The AI learned to identify and compensate for various types of motion blur, resulting in videos that were noticeably more stable and watchable than those captured with comparable phones lacking this deep learning enhancement. The model’s ability to anticipate motion was key to its success.
Image Segmentation Precision
Deep learning dramatically improved the Pixel 2’s image segmentation capabilities. Accurate segmentation is crucial for various features, including Portrait Mode, as discussed earlier. The deep learning model used for segmentation in the Pixel 2 was trained on a massive dataset of images, enabling it to identify objects and their boundaries with high precision. This allowed for more accurate background blurring, better subject isolation, and generally more refined image editing capabilities. The improvement in segmentation accuracy directly translated to better results in various camera features, enhancing the overall user experience.
Deep Learning Impact Summary
Feature | Improvement via Deep Learning | Example | Result |
---|---|---|---|
Super Res Zoom | Enhanced detail and sharpness at higher zoom levels | Zooming in on a distant building | Clearer image with minimal pixelation |
Portrait Mode Bokeh | Accurate subject segmentation and background blur | Portrait photo with complex hair | Natural-looking bokeh, sharp subject |
Video Stabilization | Smoother videos even with significant camera movement | Recording video while walking | Stable video with minimal shake |
Image Segmentation | More precise object and boundary identification | Separating a person from a busy background | Clean separation for editing or special effects |
Limitations and Future Improvements
The Google Pixel 2, while revolutionary for its time, showcased the nascent power of deep learning in mobile photography. However, even groundbreaking technology has its limitations. Understanding these limitations is crucial not only for appreciating the Pixel 2’s achievements but also for charting the course of future advancements in mobile image processing.
Deep learning models, particularly those running on the limited processing power of a smartphone, face inherent constraints. The Pixel 2’s computational photography relied heavily on these models, and consequently inherited some of their shortcomings. These limitations, while understandable given the technological landscape of 2017, highlight areas ripe for improvement in subsequent generations of mobile devices.
Computational Demands and Power Consumption
The Pixel 2’s deep learning models, while optimized, still demanded significant processing power. This translated to increased battery drain, a common complaint among users. More complex models, aiming for even higher image quality, would exacerbate this issue. Future improvements could involve developing more efficient neural network architectures, such as MobileNets, designed specifically for low-power devices. Techniques like model quantization and pruning could also reduce the model’s size and computational needs without significantly impacting performance. For example, reducing the precision of the model’s weights from 32-bit floating-point to 8-bit integers could drastically reduce memory usage and computation time. This trade-off between accuracy and efficiency is a key area of ongoing research.
Performance in Low-Light Conditions
Even with HDR+ and deep learning enhancements, the Pixel 2 struggled in extremely low-light situations. Noise reduction algorithms, while effective in many scenarios, could sometimes lead to over-smoothing, resulting in a loss of detail. Future advancements could involve incorporating more sophisticated noise models within the deep learning pipeline, perhaps incorporating techniques like denoising autoencoders or generative adversarial networks (GANs) trained on vast datasets of low-light images. Imagine a system that not only reduces noise but also intelligently reconstructs lost details, creating images that are both clean and rich in texture. This would require advancements in both hardware and software, with more powerful image sensors and more efficient deep learning algorithms working in tandem.
Generalization and Robustness
Deep learning models are trained on specific datasets, and their performance can degrade when presented with images significantly different from those in the training set. The Pixel 2’s camera might have performed exceptionally well on certain types of scenes, but it might have struggled with others, such as those with unusual lighting conditions or complex compositions. Future improvements should focus on improving the robustness and generalization capabilities of these models. Techniques like data augmentation (artificially expanding the training dataset) and adversarial training (making the model robust to noisy or adversarial examples) could help address this limitation. Imagine a camera that consistently delivers high-quality images regardless of the scene’s complexity or lighting conditions. This is a significant challenge, but one that is actively being addressed by the research community.
Potential Research Directions for Improving Mobile Camera Deep Learning
The pursuit of superior mobile photography through deep learning is an ongoing journey. Several research directions promise significant advancements:
- Developing more efficient deep learning architectures tailored for mobile hardware.
- Improving the robustness and generalization capabilities of deep learning models for mobile cameras.
- Exploring novel deep learning techniques for handling challenging scenarios, such as low-light photography and high-dynamic-range imaging.
- Integrating deep learning with other image processing techniques, such as computational photography algorithms.
- Developing methods for optimizing deep learning models for specific camera hardware and sensor characteristics.
Illustrative Examples
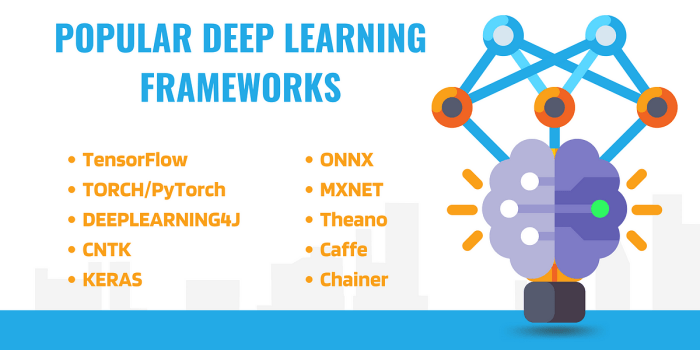
Source: medium.com
The Google Pixel 2’s deep learning prowess truly shines in challenging photographic scenarios. Let’s examine how its sophisticated algorithms tackle difficult lighting conditions and complex scenes, revealing the power behind the pixel-perfect results. We’ll explore specific examples to illustrate the impact of these models on image quality.
Consider a dimly lit street scene at night, perhaps a bustling city street with neon signs and dark alleyways. This presents a classic low-light, high-contrast challenge. Without deep learning, the image would likely be noisy, with significant grain and a loss of detail in the shadows. Colors might appear muted and inaccurate. The Pixel 2, however, leverages multiple deep learning models to address these issues. First, a dedicated low-light enhancement model analyzes the scene, identifying areas of low illumination. It then uses sophisticated algorithms to reduce noise without sacrificing detail. Simultaneously, a HDR+ model merges multiple exposures to expand the dynamic range, capturing details in both the bright neon signs and the dark recesses. A separate model refines color accuracy, ensuring the final image is vibrant and true to life, even in challenging lighting.
Low-Light Image Enhancement
Imagine a photograph of a dimly lit restaurant interior. Without the Pixel 2’s deep learning noise reduction model, the image would be significantly grainy, with noticeable artifacts. Colors would appear dull and washed out. The details in the shadows – perhaps the texture of a tablecloth or the intricate carvings on a piece of furniture – would be lost in the noise. With the deep learning model active, the image is dramatically improved. The noise is significantly reduced, revealing a much cleaner and sharper image. Colors become more vibrant and accurate. The details in the shadows are now clearly visible, adding depth and richness to the overall scene. The difference is striking; the image with the deep learning model applied possesses a clarity and detail that is simply unattainable without it. The algorithm intelligently distinguishes between actual image details and noise, preserving the former while eliminating the latter. This is achieved through a complex process of analyzing image patterns and textures, learning from vast datasets of images, and applying sophisticated filtering techniques.
Impact of Noise Reduction Model
Let’s focus on the visual impact of the noise reduction model specifically. Imagine a close-up shot of a textured surface, like a piece of rough-hewn wood. In an image processed without the noise reduction model, you would see a significant amount of digital noise, appearing as small, randomly distributed speckles throughout the image. These speckles obscure the underlying texture of the wood, making it appear blurry and indistinct. Applying the deep learning noise reduction model significantly reduces these speckles, revealing the intricate details of the wood grain. The texture becomes sharp and clear, revealing the individual grooves and variations in the wood’s surface. The color also appears richer and more accurate, as the noise reduction doesn’t just remove the speckles but also helps to refine color information. The overall impression is one of significantly improved clarity and detail.
Last Word: Google Deep Learning Model Pixel 2
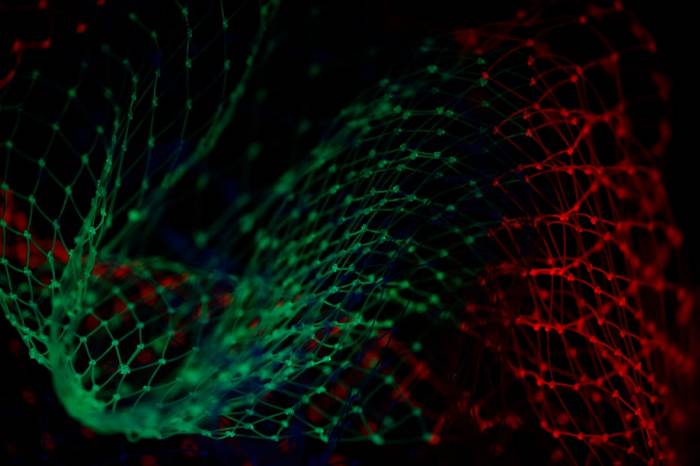
Source: medium.com
The Google Pixel 2’s camera wasn’t just a leap forward in smartphone photography; it was a showcase of deep learning’s potential. By cleverly leveraging AI, Google created a camera experience that still holds up today. While limitations existed, the innovations laid the groundwork for future advancements in mobile imaging. The Pixel 2’s legacy? Proving that AI could transform how we capture and experience the world, one stunning photo at a time.