Advertisement
Waymo self driving tech avoid uber accident – Waymo self-driving tech avoided Uber accident – a stark contrast highlighting the critical differences in safety protocols and technological sophistication. The tragic Uber incident underscored the inherent risks of autonomous vehicles, prompting a closer look at Waymo’s approach, which boasts a significantly more robust sensor suite, advanced mapping capabilities, and a layered safety system involving both automated and human oversight. This deep dive examines the technological chasm between the two companies, exploring how Waymo’s design might have prevented the devastating Uber accident.
We’ll dissect Waymo’s core technology, from its LiDAR and camera systems to its sophisticated mapping and localization algorithms. Then, we’ll analyze the Uber accident in detail, identifying potential technological failures and comparing the safety protocols of both companies. Finally, we’ll explore hypothetical scenarios and discuss future improvements in autonomous vehicle technology, focusing on how machine learning and AI can enhance safety and prevent future tragedies.
Waymo’s Technology Overview
Waymo’s self-driving technology represents a significant leap forward in autonomous vehicle development. It’s not just about avoiding accidents; it’s about creating a system capable of navigating complex real-world scenarios with a level of safety and efficiency exceeding human drivers. This overview delves into the core components that make Waymo’s approach unique.
Waymo’s self-driving system is built upon a sophisticated interplay of hardware and software, working in concert to perceive the environment, plan a route, and execute driving maneuvers safely and efficiently.
Sensor Suite
The Waymo vehicle’s perception capabilities rely on a diverse sensor suite designed for redundancy and robustness. This multi-sensor approach allows the system to build a comprehensive understanding of its surroundings, even in challenging conditions like low light or inclement weather. The core components include: LiDAR, providing a 3D point cloud representation of the environment; cameras, offering high-resolution visual data for object recognition and scene understanding; and radar, which detects objects and their velocities, particularly useful in adverse weather. The combination of these sensors offers a more complete and reliable picture than any single sensor could provide on its own. For instance, LiDAR excels at detecting distant objects and precise distances, while cameras provide detailed visual information for object classification. Radar, impervious to weather conditions, complements the data from LiDAR and cameras to offer a complete view.
Mapping and Localization, Waymo self driving tech avoid uber accident
Waymo’s approach to mapping involves creating highly detailed 3D maps of the environment using its fleet of vehicles equipped with sensor suites. These maps are not simply static representations; they are constantly updated with real-time data collected from driving, incorporating changes in infrastructure, traffic patterns, and even temporary obstructions like construction zones. Localization, the process of determining the vehicle’s precise location within the map, is crucial. Waymo uses a combination of GPS, inertial measurement units (IMUs), and sensor data to pinpoint its position with exceptional accuracy. This precise localization is critical for safe and efficient navigation. Consider a scenario where a slight error in localization could lead to a collision with a pedestrian; Waymo’s high-precision localization significantly mitigates this risk.
Comparison to Other Autonomous Driving Systems
While many companies are pursuing autonomous driving technology, Waymo distinguishes itself through several key aspects. Firstly, Waymo’s extensive mapping and data collection efforts have resulted in exceptionally detailed and comprehensive maps covering large geographical areas. Secondly, Waymo’s reliance on a multi-sensor approach provides greater redundancy and robustness compared to systems that rely heavily on a single sensor type. Finally, Waymo has amassed a significantly larger amount of real-world driving data than most competitors, allowing for continuous improvement and refinement of its algorithms through machine learning. This vast dataset enables Waymo’s AI to handle a broader range of unpredictable scenarios, improving the overall safety and reliability of the system. For example, Waymo’s system might be trained on millions of instances of pedestrian behavior, giving it a much greater capacity to anticipate and react to unpredictable pedestrian actions compared to systems trained on smaller datasets.
The Uber Accident
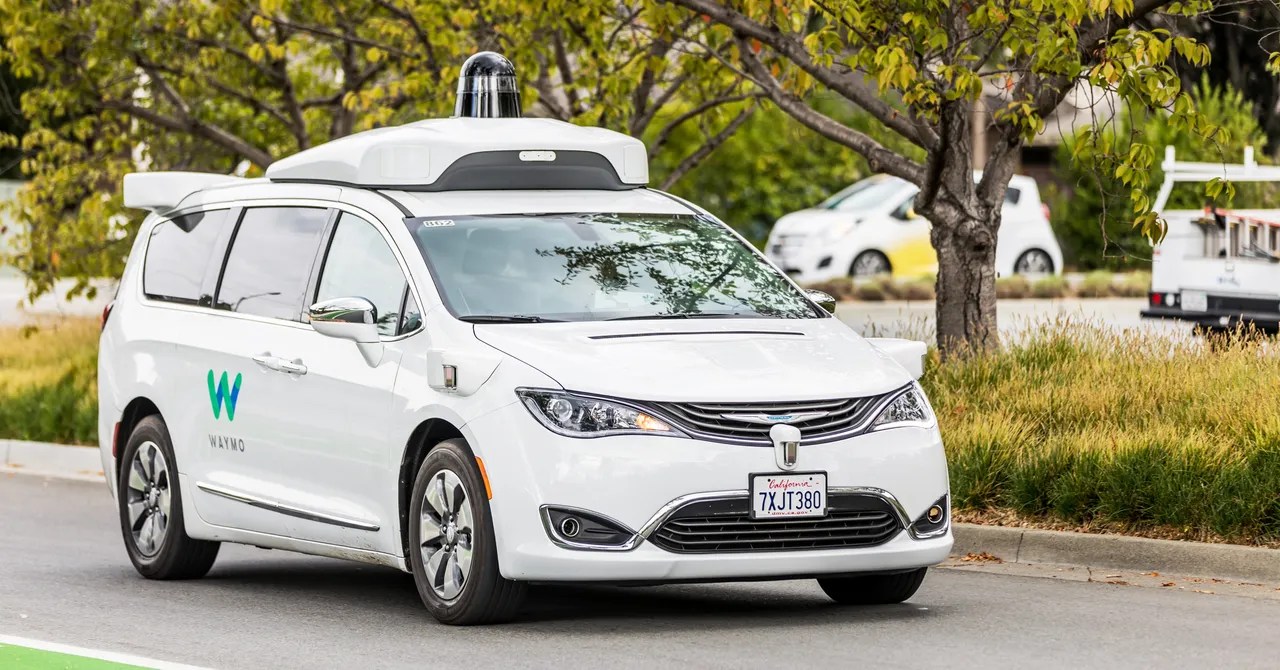
Source: wired.com
The tragic death of Elaine Herzberg in Tempe, Arizona, in March 2018, marked a watershed moment for the autonomous vehicle industry. This accident, involving an Uber self-driving Volvo SUV, brought the complexities and potential dangers of this emerging technology into sharp focus, sparking intense debate about safety protocols, regulatory oversight, and the ethical implications of deploying self-driving cars on public roads. The incident wasn’t just a setback; it served as a stark lesson in the challenges of transitioning to a fully autonomous future.
The accident occurred at night, with Herzberg pushing her bicycle across a four-lane road outside of a designated crosswalk. The Uber vehicle, operating in autonomous mode with a safety driver behind the wheel, detected Herzberg but failed to brake in time, resulting in a fatal collision. The subsequent investigation revealed a confluence of factors contributing to the tragedy, highlighting the limitations of the technology at the time and the crucial need for robust safety measures.
Technological Failures Contributing to the Uber Accident
The investigation identified several technological shortcomings. The system’s object detection and classification algorithms struggled to accurately identify Herzberg as a pedestrian, particularly in low-light conditions. Furthermore, the decision-making process within the autonomous system, concerning braking and evasive maneuvers, was found to be inadequate. While the safety driver was present, the system’s delay in alerting the driver, coupled with the driver’s inattention, meant timely intervention wasn’t possible. The accident underscored the need for more sophisticated sensor fusion techniques, improved object recognition capabilities, and more reliable decision-making algorithms that can handle unpredictable situations and edge cases. The Uber system, it seems, was not prepared for this specific scenario, showcasing the inherent difficulties in programming for every possible contingency on public roads.
Regulatory and Ethical Implications of the Uber Accident
The Uber accident raised serious questions about the regulatory framework governing the testing and deployment of autonomous vehicles. The lack of clear, consistent, and comprehensive regulations at the time left a significant gap in safety oversight. The accident fueled the debate about the appropriate level of testing required before widespread deployment, the need for stringent safety standards, and the role of government agencies in ensuring public safety. Ethically, the accident forced a conversation about the responsibility of technology developers, manufacturers, and regulators in mitigating risks and ensuring that autonomous systems are deployed responsibly. The question of algorithmic bias, particularly regarding the identification of pedestrians in diverse contexts, also came to the forefront. The incident highlighted the need for a robust ethical framework guiding the development and implementation of autonomous driving technology.
Comparison of Waymo and Uber Safety Protocols
At the time of the accident, Waymo and Uber employed different approaches to safety. Waymo, for example, had a more conservative approach to testing, focusing on controlled environments and gradually expanding into more complex scenarios. Their systems emphasized a multi-layered safety approach, incorporating redundant sensors and fail-safes. In contrast, Uber’s approach at the time seemed more aggressive, with a quicker deployment strategy and potentially less emphasis on rigorous testing in diverse and challenging conditions. While both companies utilized safety drivers, the level of driver training and the system’s ability to alert the driver effectively differed significantly. Waymo’s emphasis on comprehensive testing and a layered safety approach, contrasted with Uber’s more rapid deployment, ultimately resulted in vastly different outcomes regarding safety and public perception. The accident served as a cautionary tale, highlighting the importance of a thorough and cautious approach to the development and deployment of autonomous vehicle technology.
Waymo’s Safety Mechanisms
Waymo’s self-driving technology isn’t just about getting from point A to point B; it’s about doing so safely and reliably. Their system relies on a multi-layered approach to safety, combining advanced sensor technology, sophisticated algorithms, and human oversight to minimize the risk of accidents. This intricate network aims to not only react to unexpected situations but also proactively avoid them.
Waymo employs a robust suite of safety features, far exceeding the capabilities of a human driver. These features work in concert to create a highly redundant and resilient system. The goal isn’t perfection—that’s unrealistic—but rather a level of safety significantly surpassing human driving capabilities, statistically speaking.
Redundancy and Sensor Fusion
Waymo’s vehicles aren’t reliant on a single sensor type. Instead, they utilize a combination of lidar, radar, cameras, and other sensors to build a comprehensive 360-degree view of their surroundings. This sensor fusion allows the system to cross-check data from multiple sources, reducing the impact of individual sensor failures. If one sensor malfunctions, others can compensate, ensuring continuous and reliable perception of the environment. This redundancy is crucial for handling unexpected events or challenging weather conditions, like heavy fog or rain, which can impair the performance of individual sensors. For example, lidar might struggle in dense fog, but radar can still provide reliable distance information. The system intelligently weighs the data from each sensor, prioritizing the most reliable information at any given moment.
Human Oversight
While Waymo aims for fully autonomous driving, human oversight remains a critical component of their safety strategy. Trained safety drivers are present in Waymo vehicles during testing and, in some cases, operational deployments. These drivers are prepared to intervene if the system encounters an unexpected or unmanageable situation. The presence of a safety driver adds an extra layer of security, acting as a backup to the autonomous system and ensuring passenger safety. Their role is not to actively control the vehicle but to monitor the system’s performance and intervene only when necessary. This intervention could involve taking over manual control of the vehicle in extreme situations or bringing the vehicle to a safe stop.
Object Detection and Classification
Waymo’s system excels at identifying and classifying objects in its environment. Sophisticated algorithms process sensor data to distinguish between pedestrians, cyclists, vehicles, and other objects, predicting their trajectories and potential interactions. This involves not only identifying the object itself but also understanding its behavior – is a pedestrian crossing the street? Is a car turning? This understanding allows the vehicle to make informed decisions about how to navigate the environment safely. The system uses deep learning models trained on massive datasets of real-world driving scenarios to continuously improve its object detection and classification accuracy. This constant learning and refinement are crucial for adapting to diverse and unpredictable environments.
Hypothetical System Failure and Response
Imagine a scenario where a construction worker unexpectedly drops a large piece of metal debris into the road directly in front of a Waymo vehicle. The system might initially misclassify the object as a less dangerous item, perhaps a discarded piece of plastic. However, the system’s safety mechanisms would likely trigger multiple responses. First, the vehicle’s emergency braking system would engage, bringing the vehicle to a rapid stop. Simultaneously, the system would re-evaluate the object using other sensor data, updating its classification and initiating an alert to the safety driver. The safety driver, observing the situation, could then take control if necessary, ensuring the safety of passengers and other road users. The event would then be thoroughly investigated to understand the cause of the initial misclassification and improve the system’s performance in similar situations.
Comparative Analysis: Waymo vs. Uber (Post-Accident): Waymo Self Driving Tech Avoid Uber Accident
The tragic Uber accident in 2018 served as a stark wake-up call for the autonomous vehicle industry. Both Uber and Waymo, key players in the field, responded with significant changes to their safety protocols. However, their approaches, driven by differing corporate cultures and technological philosophies, diverged in several key aspects. This analysis examines the post-accident safety improvements implemented by both companies, highlighting similarities and differences in their strategies.
Analyzing the responses of Waymo and Uber post-accident reveals contrasting approaches to safety. While both companies implemented changes, the scale, nature, and underlying philosophy behind these changes differ significantly. Waymo, known for its meticulous approach to data collection and analysis, prioritized incremental improvements to its existing safety systems. Uber, on the other hand, underwent a more dramatic overhaul, focusing on immediate, visible changes to regain public trust.
Waymo’s self-driving tech, a marvel of AI, expertly navigated a near-miss with an Uber vehicle, showcasing its superior safety features. It got me thinking about sophisticated technology in general; I mean, even the subtle elegance of a kindle oasis in champagne gold is a testament to smart design. Ultimately, both exemplify a focus on precision and user experience, reminding us that advanced tech is all about seamless integration and avoiding potential catastrophes, just like Waymo did that day.
Safety Protocol Changes
Feature | Waymo | Uber | Comparison |
---|---|---|---|
Safety Driver Oversight | Waymo continued to utilize safety drivers in its test vehicles, but gradually reduced their reliance as confidence in the system grew. They also focused on improving the training and responsiveness of safety drivers. | Uber initially had safety drivers, but the accident led to a temporary suspension of autonomous testing and a significant increase in the level of safety driver training and oversight. They eventually reinstated testing with a stronger emphasis on driver monitoring and intervention capabilities. | Both companies initially utilized safety drivers, but Waymo’s approach was more gradual in reducing reliance, while Uber implemented more drastic immediate changes. |
Sensor Technology and Redundancy | Waymo invested in enhancing its sensor fusion algorithms and increased redundancy in its sensor suite, aiming for a more robust perception of the environment. This involved improving the reliability and accuracy of lidar, radar, and camera data integration. | Uber also improved its sensor technology, focusing on enhanced object detection and classification, particularly for pedestrians and cyclists. However, the specifics of their improvements remain less publicly available compared to Waymo’s transparent approach. | Both companies upgraded their sensor technology, but Waymo’s focus on redundancy and public transparency stands out. |
Software and Algorithm Improvements | Waymo’s post-accident software updates focused on refining its decision-making algorithms, improving its ability to handle unexpected scenarios and edge cases. This included better prediction of pedestrian and vehicle behavior. | Uber’s software improvements were less publicly detailed, but likely included updates to object recognition and decision-making algorithms, addressing shortcomings identified in the accident investigation. | Both improved algorithms, but the extent and specifics of Uber’s changes remain less transparent. |
Testing and Validation Procedures | Waymo intensified its rigorous testing and simulation procedures, including more extensive testing in complex urban environments and edge cases to improve the system’s robustness. | Uber significantly revised its testing protocols, implementing stricter safety guidelines and increasing the scrutiny of test data analysis. The exact details of these changes are not fully public. | Both companies strengthened their testing protocols, but the level of public transparency regarding the specifics of Uber’s changes is significantly lower. |
Hypothetical Scenario: Waymo Navigating a Similar Situation
Imagine a bustling Tempe, Arizona afternoon. A Waymo vehicle, let’s call it Waymo-Alpha, is navigating a relatively busy intersection. Unlike the Uber accident, there’s no pedestrian directly in the vehicle’s path. However, a bicycle courier, distracted by their phone, suddenly swerves from a bike lane into the street directly in front of Waymo-Alpha, who is proceeding at a moderate speed through a green light. This unexpected maneuver happens too quickly for a human driver to react effectively.
Waymo’s Technological Response to the Unexpected Swerve
Waymo-Alpha’s suite of sensors – lidar, radar, and cameras – instantly detect the cyclist’s erratic movement. The vehicle’s sophisticated algorithms process this information in milliseconds, far faster than a human brain. The system immediately identifies the cyclist as a potential hazard, assessing their speed, trajectory, and proximity to the vehicle. Crucially, Waymo’s system doesn’t simply react to the cyclist’s immediate position but also predicts their likely future path, factoring in the cyclist’s apparent lack of awareness.
Step-by-Step Account of Waymo-Alpha’s Actions
1. Hazard Detection: Waymo-Alpha’s sensors detect the cyclist’s unexpected swerve.
2. Risk Assessment: The system calculates the probability of a collision, considering the cyclist’s speed, trajectory, and the vehicle’s braking capabilities. It also considers the potential for secondary collisions if an emergency stop is initiated.
3. Decision-Making: Based on the risk assessment, the system determines the safest course of action. In this scenario, a complete stop would likely be deemed the safest option, considering the immediate threat and the potential for a more severe accident if it attempted to maneuver around the cyclist.
4. Execution: Waymo-Alpha smoothly applies its brakes, initiating a controlled deceleration. The system monitors the cyclist’s movements continuously, adjusting braking force if necessary to avoid a collision.
5. Post-Incident Monitoring: After coming to a complete stop, the system logs the event, recording sensor data, system actions, and contextual information. This data contributes to the ongoing improvement of Waymo’s algorithms.
Outcome Comparison: Waymo vs. Uber
Unlike the Uber accident, which resulted in a fatality due to the driver’s failure to recognize and respond appropriately to the pedestrian’s presence, Waymo-Alpha’s actions would likely prevent a collision. The system’s superior processing speed, predictive capabilities, and integrated safety protocols would allow for a safe and controlled stop, avoiding injury to the cyclist and damage to the vehicle. The cyclist might experience a sudden stop, but the likelihood of serious injury is significantly reduced due to the controlled braking. This hypothetical scenario highlights the potential of autonomous driving technology to mitigate accidents caused by human error or unexpected events.
Future Implications and Improvements
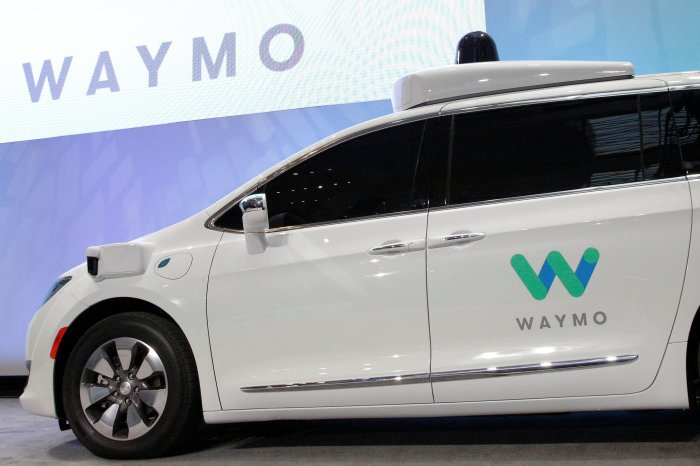
Source: wsj.net
The Uber accident, tragically avoidable with better technology, highlighted critical areas for improvement in autonomous vehicle systems. Waymo’s impressive safety record underscores its advancements, but the potential for further enhancement is immense, particularly in edge cases and unpredictable human behavior. Future iterations must prioritize not just avoiding accidents, but also gracefully handling unexpected situations with robust fail-safes and proactive decision-making.
The integration of more sophisticated AI and machine learning algorithms is paramount. This isn’t just about adding more data; it’s about smarter data processing and more nuanced interpretation of complex scenarios. Waymo’s success hinges on refining its understanding of the real world, including the unpredictable actions of pedestrians, cyclists, and other drivers. This requires moving beyond simple object detection to anticipating actions and predicting potential hazards.
Enhanced Pedestrian and Cyclist Prediction Models
Waymo’s current systems excel at detecting objects. However, predicting the erratic movements of pedestrians and cyclists – especially in crowded urban environments – remains a challenge. Improved models could incorporate factors like body language, gaze direction, and even contextual information (e.g., the presence of a crosswalk or traffic signal) to anticipate potential conflicts more accurately. This predictive capability would allow the autonomous vehicle to react proactively, slowing down or altering its trajectory before a potentially dangerous situation arises. For example, a model could identify a pedestrian who appears distracted and about to step into the road, prompting a preemptive braking maneuver. This level of anticipation is crucial for preventing accidents involving vulnerable road users.
Advanced Sensor Fusion and Redundancy
Current autonomous vehicles rely on a suite of sensors, including lidar, radar, and cameras. However, sensor failures can occur. Future improvements should focus on more robust sensor fusion algorithms that can effectively combine data from multiple sources, even if one sensor is malfunctioning. Implementing redundancy – having backup systems ready to take over in case of primary sensor failure – is equally important. This could involve using different types of sensors to gather redundant data or having multiple independent processing units capable of making driving decisions. A practical example would be having a secondary lidar system ready to take over if the primary system malfunctions, ensuring continuous and reliable environmental perception.
A New Safety Feature: Proactive Hazard Avoidance System
A hypothetical but plausible safety feature that could have potentially prevented the Uber accident is a “Proactive Hazard Avoidance System” (PHAS). PHAS would go beyond reactive braking and steering; it would actively predict and mitigate potential hazards based on a comprehensive understanding of the driving environment. This system would utilize advanced machine learning to analyze sensor data, traffic patterns, and historical accident data to identify high-risk scenarios. In the Uber accident scenario, PHAS might have identified the pedestrian’s unexpected movement and predicted a collision, initiating a preemptive maneuver—a complete stop or a sharp swerve—well before the impact. The system would continuously assess risk and adjust its response accordingly, creating a multi-layered safety net. This proactive approach contrasts with reactive systems that only respond after a hazard has already been detected.
Final Review
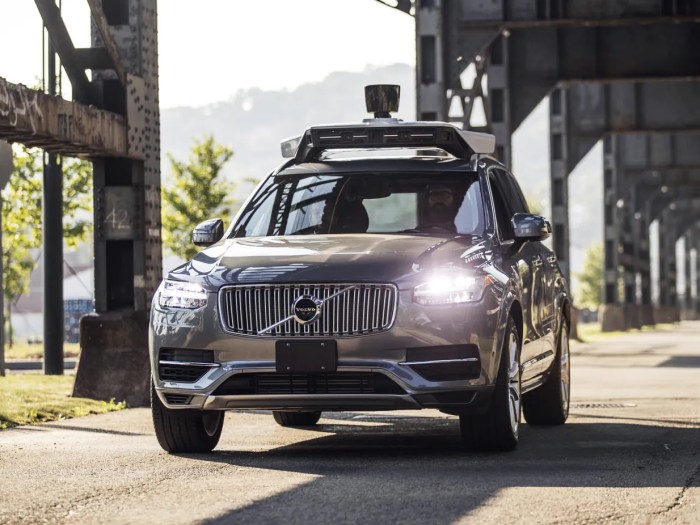
Source: wired.com
The difference between Waymo’s success in avoiding similar accidents and Uber’s tragic failure boils down to a multifaceted approach to safety. Waymo’s investment in advanced sensor technology, rigorous testing, and a layered system of human oversight proves crucial. While autonomous driving technology holds immense potential, the Uber accident serves as a stark reminder of the importance of robust safety measures. The future of self-driving cars hinges on continuous innovation, rigorous testing, and a commitment to prioritizing safety above all else. The contrast between Waymo and Uber underscores the long road ahead, but also illuminates the path toward a safer, more autonomous future.